Why the Future of Analytical Data Must Consider AI Principles
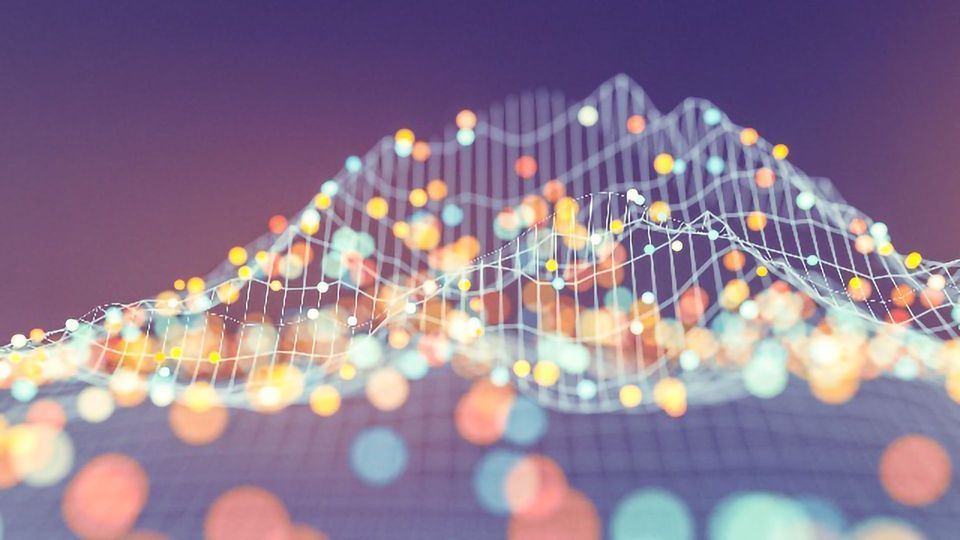
Complete the form below to unlock access to ALL audio articles.
Data science is the collection of computational methods that enable artificial intelligence. Critical strategic Initiatives in R&D over the last decade have leveraged artificial intelligence (AI) and data science. Both of these key areas require the capability for systems to manage large amounts of data efficiently. For strategic initiatives to produce value to stakeholders, data needs to be collected, stored and organized in a scalable and robust manner, to ensure it is properly structured for use. To do this, biotech and pharma organizations should:
1) Ensure that every scientist – whether synthetic, analytical, chemical, etc. – is involved in data science planning and strategy; and
2) Ensure that software-based data access is provisioned to help manage the chemically intelligent info.
Moreover, with increasing use of automation in scientific experimentation workflows, and COVID-19 driving even more interest in data science and AI, data accessibility is more important than ever. Here’s why.
Data science is for all scientists
“Without data, you're just another person with an opinion.”
-W. Edwards Deming
The scientific method relies upon the ability to collect observational data, perform analysis and justify one’s interpretation and conclusions. Technological advancements in sensors, networks and data storage have created exponential growth in the volume and variety of data that a scientist can “access” before, during and after their experiments.
For example, in many scientific and biomedical industries, chemists, biologists and materials scientists study substances at the atomic/molecular scale. These researchers apply their knowledge and experience to design reasonable experimental strategies to produce desirable outcomes.
In these processes, there are now more sources of data than ever before. In many of these experiments, particularly for “molecule-generating” processes, such as synthesis and catalysis, sensors that measure “process attributes” (e.g., temperature, time, pressure and composition) throughout experimentation provide scientists with insights that will allow for route selection, optimization and process robustness. Researchers then use this sensor data to make decisions about materials, processes and next steps. To take full advantage of this data, it should be fed into AI algorithms. However, there are several key challenges when looking to use the information generated by this variety of sensors as training data for AI.
Data heterogeneity and orthogonality
One use of sensor data is to track experiment progress. For sensors that confirm a substance’s “quality attributes,” like identity and composition, resultant data generated is usually from a variety of analysis instrumentation. The formats and digital structure of, for example, multidimensional NMR (for identity confirmation) and LC/MSn (for composition), presents a significant challenge for data science systems. For each analysis technique, systems must support an exhaustive set of digital formats, and include application logic to interpret relevant data “features.” Moreover, usually interpretation requires the inclusion of orthogonal data: specifically, sample and experiment metadata, related chemical/biological substance data and specification acceptance data.
Data volume and frequency
The volume of data that sensors (and therefore instruments) can generate and the frequency of sampling are also a challenge for data science systems. Should scientists require compositional analysis over a period of time to effectively characterize a process, the volume of data is considerable. For example, a one-hour reaction is sampled every five minutes, resulting in twelve full-scan, accurate mass LC/MSn experiments. For this single longitudinal study, the supporting system would require 12GB of storage space. When projecting the use of such data across an institution, data volume and corresponding performance requirements for AI related system functions must be carefully considered.
Data assembly and semiotics
The traditional means by which multi-attribute analysis experiments are “summarized” (and correspondingly supported by digital systems) is that they are reduced to tabular, textual, numerical and/or image abstractions.
Institutions and organizations should invest in systems that address the challenges of data abstraction. The ability to glean relevant process insights allows for facile, fully informed decision making – with the possibility of identifying “latent” factors – those factors that contribute to experimental outcomes but may not have been anticipated in advance.
Figure 1: LC/MSn data shows the value of visual plots. Credit: ACD/Labs
Moreover, the representation of analysis results can be enhanced by utilizing relevant visual plots. In the case of LC/MSn data (see figure 1), three interactive plots containing 2D chromatogram plots, with each “feature” (in this case detected and characterized peaks) linked to the corresponding MS1 and MS2 Plots (where MS channel plots are linked by precursor ion values). Certainly, this allows for easier human review; but additional value from such visual representation, which is called semiotics, can also allow for machine-based image analysis to be used.
Looking to the future
As data science initiatives proliferate across institutions, stakeholders concerned with utilizing analytical results should consider the following key capabilities:
- Analytical data engineering: ability to extract and transform the exhaustive set of analytical data from relevant instrumentation, accounting for various “data assembly” requirements. The data structures post-transformation should allow for both human and machine-based accessibility/usage.
- Analytical data management: ability to store resultant datasets in accordance with relevant data integrity principles.
- System integration: ability to integrate data management to related systems: ELN, LIMS, substance registration systems and lab execution systems.
- Data visualization: ability to present relevant display of analysis datasets for both human and machine usage.
Conclusion
As institutions seek to automate various experimental “operations” the volume and variety of data generated will only continue to increase in scale. Stakeholders responsible for implementing and ultimately utilizing data science should carefully consider how to best account for this (increasing) volume and variety of analytical datasets.
About the author: Andrew Anderson is VP Innovation & Informatics Strategy at ACD/Labs