Understanding Structural Biology, Its Applications and Creating a Molecular Model
In this article, we explore what structural biology tells us and how techniques can be applied and combined.
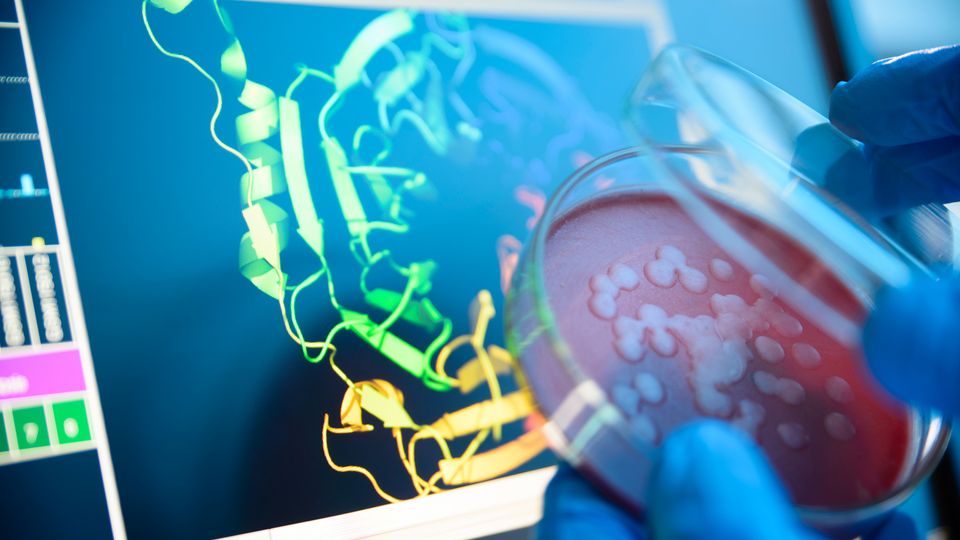
Complete the form below to unlock access to ALL audio articles.
An Introduction to Structural Biology. Credit: Technology Networks via YouTube
What is structural biology?
Structural biology is a field dedicated to the study of the structure of molecules that form living matter, including proteins, nucleic acids, lipid membranes and carbohydrates. The information provided by structural biology is crucial to understand the function and dynamics of biomolecules, as well as the mechanisms of interaction among them.1
What can structural biology tell us?
- Macromolecule structure
- Primary structure, secondary structure, tertiary structure and quaternary structure
- Structural domains
- Structural motifs
- Protein folding
- Protein shape
- Protein dynamics
Common techniques in structural biology
Integrative structural biology
In-silico protein structure predictions
Applications of structural biology
- Identifying drug targets
- Studying host–pathogen interactions
- Protein aggregation in health and disease
- Determining virus structure
- Making a molecular model
The origins of modern structural biology date back to the 1910s, when the German physicist Max von Laue discovered that biological crystal samples irradiated with X-rays produced diffraction patterns that depended on their internal atomic organization.2 This discovery, together with the work of William and Lawrence Bragg, marked the beginning of the development of one of the main structural biology techniques: X-ray crystallography.3 These advances allowed the elucidation of a protein structure for the first time4 and the identification of the DNA double-helix in the 1950s.5 Nuclear magnetic resonance (NMR) spectroscopy contributed enormously to the development of structural biology from the 1980s, when scientists started to apply it extensively to the study of biomolecules.6 Finally, in the last decades, other techniques have gained prominence in structural biology, such as small-angle X-ray scattering (SAXS),7 electron paramagnetic resonance spectroscopy (EPR)8 and, especially, cryogenic electron microscopy (cryo-EM).9
What can structural biology tell us?
Structural biology provides information about the organization of living matter, including the spatial disposition of atoms and molecules, the global folding of a biopolymer or even the association of biological macromolecules to form large, intricate complexes. Additionally, structural biology is capable of unveiling details about molecular interactions and dynamic parameters, such as molecular flexibility.
Proteins and nucleic acids are the most studied biomolecules due to the large number of diverse functions they perform in living organisms. These functions are closely related to their structural organization. To address this relationship between structure and function, structural biology makes use of some fundamental concepts related to the hierarchical assembly of proteins and nucleic acids (such as macromolecule structure; primary, secondary, tertiary and quaternary structures; structural domains and motifs; protein shape), or to the process of structural organization itself from a thermodynamic point of view (protein folding and protein dynamics). These concepts are explained below.
Tertiary structure of protein
The tertiary structure of a protein is its overall shape, including the arrangement of its constituent polypeptide chains, in three-dimensional (3D) space. This 3D arrangement will be influenced by interactions between different parts of the chain(s), such as hydrogen and ionic bonds and hydrophobic interactions between nonpolar amino acid side chains.
Macromolecule structure
Macromolecules are usually considered to be biopolymers with a mass larger than 5,000 Da and are a central constituent of living matter.1 The disposition of the different atoms within the molecule and their connectivity determine the location of chemical groups able to establish interactions or to undergo chemical transformations. Their positionings are essential for the macromolecule to associate to other partners or to perform a biological activity.
Primary structure, secondary structure, tertiary structure and quaternary structure
Proteins are polymeric molecules consisting of monomers called amino acids, which are connected by peptide bonds. There are 20 different common amino acids in proteins that differ in their side chains and therefore show different physicochemical properties. The protein primary structure is defined by the distribution or sequence of amino acids in the polymer chain (Figure 1). Within the protein polymer, the angles formed by the bonds between an amino acid, the preceding one and the successive one, are called “dihedral angles” (represented as φ and ψ, respectively). These angles are defined by the non-covalent interactions established between different amino acids. When a polypeptide sequence contains a series of consecutive amino acids displaying the same dihedral angles, the polymeric chain forms regular structures known as protein secondary structures (Figure 1). The most common are α-helices (φ = -60°; ψ = -45°), β-strands (φ = -135°; ψ = 135°) and turns (variable φ and ψ values). The way in which the secondary structure elements of a protein, together with unstructured regions, fold to form a packed entity is known as the tertiary structure (Figure 1). To perform their function, many proteins need to form complexes by associating with other proteins. Each protein within the complex is a subunit and the way the different subunits associate to form a larger, organized, functional entity is known as the quaternary structure (Figure 1).1, 10, 11, 12
The structure of nucleic acids can be described following a similar scheme, as they are polymeric macromolecules composed by monomers (nucleotides) connected by phosphodiester bonds. There are five different types of nucleotides (adenine, cytosine, guanine, thymine – only in DNA – and uracil – only in RNA) and, as in the case of proteins, the specific sequence of the nucleotides defines the primary structure of nucleic acids (Figure 1). In both DNA and RNA, secondary structure elements are determined by the interactions between nucleotides, which can be sequential (stacking interactions) or non-sequential (hydrogen bonds) (Figure 1). Regarding DNA, hydrogen bonds between nucleotides from different chains generate helical structures. In the case of RNA, the most common elements of the secondary structure are helices (also called stems, formed by paired nucleotides) and loops (formed by unpaired nucleotides). DNA organizes at a higher level, forming double-helical structures, when two DNA molecules have their nucleotides paired through hydrogen bonds. This is the tertiary structure of DNA (Figure 1) and it can display diverse conformations, such as A-DNA, B-DNA (predominant in living cells) and Z-DNA. Other tertiary structures observed in DNA are triple and quadruple helices (triplexes and quadruplexes). In the case of RNA, the most common tertiary structure elements are the stem-loop (a base-paired helix that ends in a small loop) and the pseudoknot (a stem-loop in which the unpaired loop pairs with another region of the sequence). Quaternary structure (Figure 1) is the higher level of organization in nucleic acids. For DNA, it can be applied to the association with proteins (histones) to form chromatin. For RNA, a typical example is the association of various RNA molecules (subunits) to form larger entities, such as the ribosome.1, 10, 11, 12
Structural domains
In proteins, a structural domain is defined as a region of the polypeptide chain composed by less than 200 amino acids and usually capable of maintaining their folding when isolated from the rest of the protein. Structural domains are very compact and often display a globular shape. Regarding their secondary structure elements, structural domains can be classified into five groups: α (only α-helices), β (only β-strands), α/β (β-strands connected by α-helices), α+β (separate helical and β-strand regions) and cross-linked (elements of secondary structure stabilized by metal ions or disulfide bonds).1, 10, 11, 12
Structural motifs
In both proteins and nucleic acids, secondary structures can pack with each other, creating a more compact structural level (supersecondary structure) whose elements are known as structural motifs and that can be found in different molecules. In proteins, the most common structural motifs can be divided into α-packings (such as the helix-turn-helix motif), β-packings (such as the β-hairpin, β-meander, Greek key, β-barrel and β-helix) or αβ-packings (such as the αβ-barrel, Rossmann fold and βαβ motif). In the cases of nucleic acids, structural motifs are considered tertiary structure elements and some examples include the stem loops, quadruplexes, cruciform DNA and displacement loops (D-loops).1, 10, 11, 12, 13
Figure 1: Hierarchical organization of the structure of proteins and nucleic acids showing examples of the most common structural elements. Credit: Technology Networks.
Protein folding
Protein folding is a process in which a polypeptide chain adopts its functional three-dimensional (3D) molecular arrangement (called native conformation). This process takes place in a hierarchical manner, meaning that the simpler structural elements are formed first (secondary structure) and then they pack with each other to create a folding core. Once this core is constructed, global protein folding occurs in a cooperative manner. During the course of folding, many physicochemical interactions play key roles, such as hydrogen bonds, van der Waals forces and hydrophobic effects. Sometimes, special types of proteins called chaperones assist the process, ensuring correct protein folding .14
Due to the high complexity of polypeptides, the folding process could be expected to be slow, as there is a myriad of possible folding schemes. However, in reality, most proteins are able to acquire their functional structure within a time range in the order of milliseconds.15 To explain this fact, protein folding is usually illustrated with an energy landscape funnel (Figure 2). Initially, the polypeptide folding is not energetically favored (it is high energy), but once some regions of the chain start to adopt regular conformations, the energy of the folding intermediate decreases. In this context, the polypeptide chain passes through a series of intermediate steps (molten globule) that gradually decrease its energy requirement, making the process more energetically favorable. In each folding step, only a certain number of energy states are accessible and this way, the folding process becomes more and more cooperative until reaching the final structure (native structure), which has the lowest energy.16, 17
Figure 2: Energy landscape funnel showing the protein folding process from the unfolded state to the native state (lowest energy). Credit: Thomas Splettstoesse, reproduced under the Creative Commons Attribution-Share Alike 3.0 Unported license.
Protein shape
The tertiary structure of a protein determines its global shape, which is strongly related to the protein function. Many scientists have tried to develop bioinformatics tools able to classify proteins according to their shape or global structure. These tools will enable a better understanding of protein functions and the design of new proteins with specific functions for clinical or biotechnological applications.18, 19, 20
Generally, proteins can be divided into four classes regarding their global shape: globular proteins, fibrous proteins, membrane proteins and intrinsically disordered proteins (IDPs), shown in Figure 3. Globular proteins display a spheroidal shape, they can show a high degree of structural complexity and perform diverse functions. On the other hand, fibrous proteins have elongated shapes, are relatively simple from a structural point of view and play structural roles, typically constituting fibrillar networks. Membrane proteins contain regions that are able to embed into the hydrophobic core of lipid bilayers and are specialized in signal transduction or membrane trafficking. Finally, IDPs are proteins with a very low level of structuration and thus very dynamic.12, 21, 22, 23, 24
Figure 3: Examples of the different protein shapes found in nature: A) Globular (human hemoglobin, PDB: 1SI4); B) Fibrous (human keratin, PDB: 6EC0); C) Membrane protein (human CCR5 chemokine receptor, PDB: 4MBS); D) Intrinsically disordered protein (human TDP43, PDB: 2N4P). Credit: All structures are from the RCSB Protein Data Bank, reproduced under the CC0 1.0 Universal (CC0 1.0) Public Domain Dedication. A) Sen U, Dasgupta J, Choudhury D, Datta P, Chakrabarti A, Chakrabarty SB, Chakrabarty A, Dattagupta JK, B) Eldirany SA, Lomakin IB, Bunick CG, C) Tan Q, Zhu Y, Han GW, Li J, Fenalti G, Liu H, Cherezov V, Stevens RC, GPCR Network (GPCR), Zhao Q, Wu B, altered to include parallel lines to indicate the position of the membrane, D) Mompean M, Romano V, Pantoja-Uceda D, Stuani C, Baralle F, Buratti E, Laurents DV.
Protein dynamics
Proteins are not rigid solids but very dynamic macromolecules with different degrees of freedom. At the local level, rotation around chemical bonds, especially in amino acid side chains, is common and hence many chemical groups are able to modify their orientation within the protein. At a higher level, the protein backbone is flexible in those regions that are not regularly structured, such as loops or disordered regions. Rearrangement of those regions often occurs upon binding of other molecules or changes in the environmental conditions. Sometimes, this kind of structural reorientation leads to more drastic changes in the whole protein shape, with notable displacement of protein domains.25, 26, 27
As a result of these dynamic features, a protein does not display a unique structure under physiological conditions, but rather fluctuates between many different conformations (conformers). Dynamic changes in proteins are essential for function because they allow them to adapt their shape to other binding partners or receptors, or switch between active and inactive forms.25, 26, 27
Common techniques in structural biology
There are many techniques used to address structural biology questions. All these techniques possess strengths and limitations and thus, scientists usually combine some of them to achieve a more complete description of the studied system. The main techniques used in structural biology are:
- Cryo EM
- X ray crystallography (including serial femtosecond crystallography (SFX) and high-throughput (HT) crystallography)
- NMR spectroscopy
- Cross-linking mass spectrometry (XL-MS)
- Small-angle X-ray scattering (SAXS)
- Neutron diffraction
- Proteolysis
- Circular dichroism (CD)
- Electron paramagnetic resonance spectroscopy (EPR)
To find out more about the techniques used in structural biology, their strengths and limitations, visit the article below.
Integrative structural biology
Structural characterization of biomolecules is frequently challenging. While sometimes it is possible to build high-resolution molecular models, many biological molecules display characteristic features that hamper structural analysis. For this reason, integrative structural biology has emerged as a clever approach to circumvent those difficulties. This field addresses the structural study of biomolecules by integrating information obtained through different experimental techniques combined with physical and statistical methodologies.28, 29, 30, 31, 32
Integrative structural biology provides a very complete description of biomolecular ensembles by combining information about aspects such as atomic spatial organization, relative disposition of subunits in complexes, molecular dynamics and flexibility and intra- and inter-molecular interactions, obtained by different techniques. This information is transformed into structural restraints that must be fulfilled with an acceptable tolerance by molecular models that are usually built and optimized by computational methods. This process is known as integrative/hybrid (I/H) modeling and is performed in a series of consecutive and iterative steps, including the retrieval of all the available data of the system, the use of that information to build models of the structural components, the scoring and filtering of those models and, finally, the validation of the whole model.28, 29, 30, 31, 32
Integrative structural biology is an advantageous approach to overcome the inherent limitations of using a sole technique to construct a detailed structural description of a biomolecule. In this regard, the approach is especially useful to tackle the study of large, intricate biomolecular ensembles. An emblematic example of the use of I/H modeling is the structural study of the nuclear pore complex of yeast (NPC). NPC is a huge ensemble of proteins (> 500 subunits) embedded in the nuclear envelope that is the main component responsible of the transport of proteins and nucleic acids between the nucleus and the cytoplasm. Due to its size and flexibility, it is impossible to elucidate its structure using uniquely atomic resolution techniques such as X-ray crystallography or NMR spectroscopy. Nonetheless, a precise model has been solved using an integrative approach that combines information from X-ray crystallography (isolated subunit structures), XL-MS (distance restraints), quantitative MS and in vivo calibrated imaging (stoichiometry) and cryo-EM (overall complex shape). By combining the information from all of these techniques, a series of molecular models that fulfilled the imposed restraints was built. These models were ranked by the value of a scoring function that quantifies how well the models fit to the restraints. The models with highest score were selected as they were the most consistent with the experimental data, and they were optimized by computational tools that introduced slight changes in the structure without violating any restraint in order to minimize the energy of the system. Finally, to validate the overall model, it is useful to keep some experimental data aside and, once the model is built, employ these data to check whether the model is consistent with them (Figure 4).28, 29, 30, 31, 32
Figure 4: Summary of the integrative approach for structure determination. Credit: Technology Networks, modified from Rout et al.29
In-silico protein structure predictions
Proteins play a central role in every biological process, performing a wide variety of functions. These functions are closely related to their native structure. For this reason, one of the purposes of structural biology is the elucidation of protein structures to understand how they work. As explained before, solving protein structures is a challenging process that can be expensive and time consuming. In this regard, the development of increasingly powerful informatics tools has led to the expansion of the field of in-silico protein structure prediction. This discipline is focused on the inference of the 3D organization of proteins from their amino acid sequence using computational methodologies. Since proteins display a hierarchical structural organization, in-silico approaches can be applied to predict secondary, tertiary or quaternary structures. As organization complexity increases, structural prediction becomes more arduous, and it is more difficult to get a high degree of accuracy.
There are various in-silico approaches for protein structure prediction, such as secondary structure prediction, homology modeling, protein threading, ab initio methods and artificial intelligence (AI) methods.
- Secondary structure prediction (SSP): This method predicts secondary structure elements (α-helices, β-strands and turns) from the amino acid sequence by using algorithms based on machine learning, neural networks (NNs) and sequence alignments. Although SSP only gives information on the number, type and sequential position of secondary structure elements, it is a very useful and quick tool, able to reach an accuracy of around 90%.33 Some examples of software used for secondary structure prediction are PredictProtein,34 GOR35 and Jpred.36
- Homology modeling: In this method, the structure of a protein is predicted from its amino acid sequence and the use of a template structure of a homologous protein to build the model. This method provides a good accuracy provided that the sequence identity is higher than 50%. Homology modeling is limited by the availability of solved structures of homologous proteins with a significant degree of sequence identity. Examples of homology modeling tools are SWISS-MODEL,37 IntFOLD38 and ESyPred3D.39
- Protein threading: When the target protein has no homologous proteins with solved structures, its structure can be predicted using proteins with the same kinds of folds as templates. This methodology is carried out by selecting adequate protein structures as templates and aligning each amino acid of the protein with those templates. A scoring function allows evaluation of how well each amino acid fits to the template. By optimizing that scoring function, a model is constructed following statistical criteria. This methodology is able to generate good predictions when sequence identity between the target protein and template is low (< 25%). Some examples of protein threading software are Phyre240 and I-TASSER.41
- Ab initio methods: These methods are designed to predict the tertiary structure of a protein using solely its amino acid sequence as a starting point. This complex task requires a great computational effort, and it has been used with proteins of relatively small size. The strategy consists of starting with some candidate conformations and filtering them based on thermodynamic and energetic criteria. To facilitate the prediction, the initial candidates are sometimes built according to previous secondary structure predictions. The most renowned tool used for ab initio protein predictions is Rosetta.42
- AI methods: During the last decades, the increasing sophistication of computational technologies has permitted the implementation and optimization of AI approaches, especially those based on NNs and deep learning. From the amino acid sequence and without the need of templates, these methods are able of predict protein structures with a very high degree of accuracy.43, 44, 45 In 2018, the program AlphaFold was presented, a deep learning-based software to predict protein structures that allowed the elucidation of many challenging protein structures with a high degree of accuracy in a short period of time. Although it is considered a milestone in the field of protein structure prediction, it also has some limitations: it cannot reliably predict the structure of IDPs; it does not include predictions for post-translational modifications or co-factors and it describes only one conformation in proteins that can adopt multiple ones.46, 47
Applications of structural biology
There is a plethora of applications and potential applications for structural biology studies, let us consider a few of key areas.
Identifying drug targets
Structural biology is essential in the field of drug discovery in which the main goal is to find new chemical compounds targeting biomolecules as treatments for disease. The process of drug discovery based on structural information is known as structure-based drug discovery (SBDD). The SBDD workflow generally starts by identifying those proteins that are involved in the development of any disease. Then, the proteins are expressed, extracted and purified to be analyzed by different physicochemical techniques, such as X-ray crystallography, cryo-EM and NMR spectroscopy. Following an integrative structural biology approach, the 3D structure of the target protein is solved. Next, screening is performed on an active compounds database, including the computational docking of the compounds into the binding site of the target protein. Finally, the compounds that bind more favorably to the protein binding site are selected as hits. These hits will be evaluated experimentally to check their capacity to bind to the protein in vitro and in vivo (and eventually block its functionality) in order to select the best candidates (leads) to follow the drug development process. Those lead compounds will be slightly modified to create variants that potentially display better therapeutic properties (such as improved action, lower toxicity and better absorption).48, 49, 50, 51, 52, 53, 54, 55
Some examples of drugs discovered by using an SBDD approach are raltitrexed (treatment of HIV infection), isoniazid (treatment of tuberculosis) and STX-0119 (treatment of lymphoma). The use of structural biology is enormously advantageous because a structural model of the target proteins allows extensive screenings to be carried out by computational methods, considerably reducing experimental costs and time.48, 49, 50, 51, 52, 53, 54, 55
Studying host–pathogen interactions
Pathogens (viruses, bacteria and parasites) are capable of establishing interactions with the host, which help them to infect, multiply and survive within the host organism. The identification and characterization of host–pathogen interactions at the molecular level is crucial to understand how many diseases are transmitted and developed and, eventually, how they can be treated. In this regard, structural biology is a very powerful tool to predict and study host–pathogen interactions (as they usually involve protein–protein interactions) and characterize the structure of the biomolecules involved in those interactions.56, 57, 58
There are various approaches to address the prediction of host–pathogen interactions that can be classified into two categories: based on sequence and domain information and based on 3D structural information. Some of the methods based on sequence and domain information are:56, 57, 58
- Homology-based methods: They are based on the search of known interacting sequences in homologous proteins of different organisms. Since pathogens’ interacting proteins can undergo rapid variations to adapt to the host defenses, these methods are prone to generate a lot of false positives.
- Domain-based methods: As sequences can be variable, some methods use higher order structural data, such as protein domain structure. These structures are preserved in homologous proteins, even if the sequence changes.
- Motif and integration-based methods: Motifs are structural features simpler than domains and have been proven to be essential in host–pathogen interactions. Sometimes this structural information is combined with the sequence data in an integrative way to improve the predictions.
With regard to the approaches based on 3D structural information, the workflow includes the search of the genome of both host and pathogen to find proteins similar to known complexes and the use of available 3D structural data to assess the potential interactions.56, 57, 58.
From a general perspective, all these approaches use the available information provided by structural biology about structure and interactions as input data for AI tools, such as machine learning. These AI methodologies employ the data, together with a series of training sets (information given to the AI tool to improve its capacity to make good predictions), to predict networks of potential host–pathogen interactions that should be validated by experimental assays.56, 57, 58
Protein aggregation in health and disease
Protein aggregation is a process that takes place under certain circumstances in which IDPs or misfolded proteins accumulate and stack together, generating deposits inside the cell or in the extracellular medium. The product of protein aggregation can be amorphous aggregates, oligomers or amyloids, the last ones being the most clinically relevant. Amyloids are protein structures made up of two tightly packed long β-sheets forming long fibers. In humans, the presence of amyloid fibers is associated with the development of many diseases, some of them serious neurodegenerative conditions, such as Alzheimer’s, Parkinson’s, Huntington’s diseases and amyotrophic lateral sclerosis (ALS).59, 60
At present, there is no cure for these diseases and, unfortunately, they are fatal in the long term. For this reason, the structure of amyloids and their mechanism of formation and elongation have been the focus of many structural biology research projects during the last decades. The amyloid fiber structures have been analyzed by low- and high-resolution techniques, including CD spectroscopy, Förster resonance energy transfer (FRET), atomic force microscopy (AFM), dynamic light scattering (DLS), surface plasmon resonance (SPR), X-ray crystallography, cryo-EM and NMR spectroscopy.61, 62, 63, 64
All the structural information available on amyloid fibrils has allowed the characterization of its mechanism of aggregation and suggestion of different therapeutic structure-based strategies. Some of the therapeutic approaches are focused on the inhibition of the aggregation by using denaturants, chaperones, organic compounds or peptides. Other approaches are oriented to block the pathways that amyloids follow to cause pathogenicity.61, 62, 63, 64
Determining virus structure
Viruses are molecular assemblies with infectious capacity that are responsible for a myriad of diseases affecting humans, animals and plants. With a genome encoding very few proteins, viruses are able to infect and replicate inside living cells using a very simple machinery. Their simplicity is precisely one of their strengths, as it makes them difficult to target with drugs.
Viral capsids are made up of proteins and can display a great variety of shapes and sizes. Understanding how viruses assemble and interact with the host cells and their machinery is essential to develop effective treatments. In this context, different structural biology techniques have been used to gain structural information about viruses.
On one hand, some low-resolution techniques provide data about secondary structural elements and folding/unfolding dynamics, for instance CD spectroscopy65, 66 or fluorescence spectroscopy;65 or about subunit assembly and complex formation, like SAXS.67 On the other hand, high-resolution techniques are able to provide further structural details at the atomic level. For instance, MS can be used to identify protein subunits and their post-translational modifications, as well as the stoichiometry of the viral capsid.68 Alternatively the complete structure of viral capsids can be elucidated by solid-state NMR spectroscopy,69, 70 X-ray crystallography71 and, especially, cryo-EM.72
Structural information about viruses and their components has been used for the discovery of new antiviral drugs and for the search of potential repurposing opportunities of pre-existing drugs. The strategy followed for antiviral drug discovery is the classic SBDD approach but, in this case, the target proteins are those expressed by the viruses.73, 74, 75
Making a molecular model
Structural biology is concerned with the search for information about the structural features of biomolecules by using a plethora of physicochemical techniques. The use of experimental data from different techniques in an integrative way enables the construction of molecular models. These models are structures whose atomic organization matches with the experimental results within a tolerance range. The physicochemical techniques that supply the experimental data for molecular modeling are just as important as the computational tools (including AI resources) that help to integrate all the information and optimize models to achieve the highest accuracy.76, 77, 78, 79
The importance of molecular models stems from the fact that they are very useful to understand how biomolecules organize, their dynamics and their ability to interact with other molecules. This information is not only relevant for basic science, but also for its applications in the field of medicine, pharmacology or biotechnology.76, 77, 78, 79
Building accurate molecular models of biomolecules is a grueling task, costly and time-consuming. For this reason, scientists have created specialized public databases that are accessible on the internet where they deposit the solved structures. In this regard, the most important database is the Protein Data Bank (PDB),80 where thousands of peptide and protein structures elucidated by different techniques can be found and downloaded, as well as all the information available. There are similar databases for nucleic acids (NDB)81 and carbohydrates (CSDB).82
1. Nelson DL, Cox MM. Lehninger Principles of Biochemistry. New York: WH Freeman; 2021. ISBN:9781319322342.
2. Von Laue M, van der Lingen JS. Beobachtungen über Röntgenstrahlinterferenzen. Naturwissenschaften. 1914; 2(13):328-329. doi:10.1007/BF01495712
3. Bragg WH. The analysis of crystal structure by X-rays. Science. 1924; 60(1546):139-149. doi:10.1126/science.60.1546.139
4. Kendrew JC, Bodo G, Dintzis HM, Parrish RG, Wyckoff H, Phillips DC. A three-dimensional model of the myoglobin molecule obtained by X-ray analysis. Nature. 1958; 181(4610):662-666. doi:10.1038/181662a0
5. Watson JD, Crick FHC. Molecular structure of nucleic acids: A structure for deoxyribose nucleic acid. Nature. 1953; 171:737-738. doi:10.1038/171737a0
6. Wüthrich K. Protein structure determination in solution by NMR spectroscopy. J Biol Chem. 1990; 265(36):22059-22062. doi:10.1016/S0021-9258(18)45665-7
7. Svergun DI, Koch MHJ. Small-angle scattering studies of biological macromolecules in solution. Rep Prog Phys. 2003; 66:1735-1782. doi:10.1088/0034-4885/66/10/R05
8. Hubbell WL, Altenbach C. Investigation of structure and dynamics in membrane proteins using site-directed spin labeling. Curr Opin Struc Biol. 1994; 4:566-573. doi:10.1016/S0959-440X(94)90219-4
9. Callaway E. Revolutionary cryo-EM is taking over structural biology. Nature. 2020; 578:201. doi:10.1038/d41586-020-00341-9
10. Liljas A, Liljas L, Ash M-R, Lindblom G, Nissen P, Kjeldgaard M. Textbook of structural biology. Singapore: World Scientific Publishing; 2017. ISBN:9789813142466
11. Pal S. Fundamentals of molecular structural biology. London: Academic Press; 2020. ISBN:9780128148556
12. Sun PD, Foster CE, Boyington JC. Overview of protein structural and functional folds. Curr Protoc Prot Sci. 2004; 35(1): 17-1. doi:10.1002/0471140864.ps1701s35
13. Johansson MU, Zoete V, Michielin O, Guex N. Defining and searching for structural motifs using DeepView/Swiss-PdbViewer. BMC Bioinformatics. 2012; 13:173. doi:10.1186/1471-2105-13-173
14. Scalvini B, Sheikhhassani V, Mashaghi A. Topological principles of protein folding. Phys Chem Phys. 2021; 23:21316-21328. doi:10.1039/D1CP03390E
15. Kubelka J, Hofrichter J, Eaton WA. The protein folding ‘speed limit’. Curr Opin Struc Biol. 2004; 14:76-88. doi:10.1016/j.sbi.2004.01.013
16. Bryngelson JD, Onuchic JN, Socci ND, Wolynes PG. Funnels, pathways, and the energy landscape of protein folding: a synthesis. Proteins: Struct Funct Bioinf. 1995; 21(3):167-195. doi:10.1002/prot.340210302
17. Díaz-Villanueva JF, Díaz-Molina R, García-González, V. Protein folding and mechanisms of proteostasis. Int J Mol Sci. 2015; 16:17193-17230. doi:10.3390/ijms160817193
18. Han X, Sit A, Christoffer C, Chen S, Kihara D. A global map of the protein shape universe. PLoS Comput Biol. 2019; 15(4):e1006969. doi:10.1371/journal.pcbi.1006969
19. Magner A, Szpankowski W, Kihara D. On the origin of protein superfamilies and superfolds. Scientific Reports. 2015; 5(1):1-7. doi:10.1038/srep08166
20. Shannon G, Marples CR, Toofanny RD, Williams PM. Evolutionary drivers of protein shape. Sci Rep. 2019; 9(1):1-15. doi:10.1038/s41598-019-47337-8
21. Engel A, Gaub HE. Structure and mechanics of membrane proteins. Annu Rev Biochem. 2008; 77:127-148. doi:10.1146/annurev.biochem.77.062706.154450
22. Oldfield CJ, Dunker AK. Intrinsically disordered proteins and intrinsically disordered protein regions. Annu Rev Biochem. 2014; 83:553-584. doi:10.1146/annurev-biochem-072711-164947
23. Gruebele M, Dave K, Sukenik S. Globular protein folding in vitro and in vivo. Annu Rev Biophys. 2016; 45:233-251. doi:10.1146/annurev-biophys-062215-011236
24. Yigit S, Dinjaski N, Kaplan DL. Fibrous proteins: At the crossroads of genetic engineering and biotechnological applications. Biotecnol Bioeng. 2016; 113(5):913-929. doi:10.1002/bit.25820
25. Khodadadi S, Sokolov AP. Protein dynamics: From rattling in a cage to structural relaxation. Soft Matter. 2015; 11:4984-4998. doi:10.1039/c5sm00636h
26. Lewandowski JR, Halse ME, Blackledge M, Emsley L. Direct observation of hierarchical protein dynamics. Science. 2015; 348(6234):578-581. doi:10.1126/science.aaa6111
27. Charlier C, Cousin SF, Ferrage F. Protein dynamics from nuclear magnetic relaxation. Chem Soc Rev. 2016; 45:2410-2422. doi:10.1039/c5cs00832h
28. Masrati G, Landau M, Ben-Tal N, Lupas A, Kosloff M, Kosinski J. Integrative structural biology in the era of accurate structure prediction. J Mol Biol. 2021; 433(20):167127. doi:10.1016/j.jmb.2021.167127
29. Rout MP, Sali A. Principles for integrative structural biology studies. Cell. 2019; 177(6):1384-1403. doi:10.1016/j.cell.2019.05.016
30. Sali A. From integrative structural biology to cell biology. J Biol Chem. 2021; 296:100743. doi:10.1016/j.jbc.2021.100743
31. Ward AB, Sali A, Wilson IA. Integrative structural biology. Science. 2013; 339(6122):913-915. doi:10.1126/science.1228565
32. Srivastava A, Tiwari SP, Miyashita O, Tama F. Integrative/hybrid modeling approaches for studying biomolecules. J Mol Biol. 432(9):2846-2860. doi:10.1016/j.jmb.2020.01.039
33. Ho C-T, Huang Y-W, Chen T-R, Lo C-H, Lo W-C. Discovering the ultimate limits of protein secondary structure prediction. Biomolecules. 2021; 11:1627. doi:10.3390/biom11111627
34. Bernhofer M, Dallago C, Karl T, Satagopam V, Heinzinger M, Littman M, et al. PredictProtein – Predicting protein structure and function for 29 years. Nuc Ac Res. 2021; 49:W535-W540. doi:10.1093/nar/gkab354
35. Kloczkowski A, Ting K-L, Jernigan RL, Garnier J. Combining the GOR V algorithm with evolutionary information for protein secondary structure prediction from amino acid sequene. Proteins: Struct Funct Genet. 2002. 49:154-166. doi:10.1002/prot.10181
36. Drozdetskiy A, Cole C, Procter J, Barton GJ. JPred4: A protein secondary structure prediction server. 2015; Nuc Ac Res. 43:W389-W394. doi:10.1093/nar/gkv332
37. Waterhouse A, Bertoni M, Bienert S, Studer G, Tauriello G, Gumienny R, et al. SWISS-MODEL: Homology modelling of protein structures and complexes. Nuc Ac Res. 2018; 46:W296-W303. doi:10.1093/nar/gky427
38. McGuffin LJ, Adiyaman R, Maghrabi AHA, Shuid AN, Brackenridge DA, Nealon JO, et al. IntFOLD: An integrated web resource for high performance protein structure and function prediction. Nuc Ac Res. 2019; 47:W408-W413. doi:10.1093/nar/gkz322
39. Lambert C, Léonard N, De Bolle X, Depiereux E. ESyPred3D: Prediction of proteins 3D structures. Bioinformatics. 2002; 18(9):1250-1256. doi:10.1093/bioinformatics/18.9.1250
40. Kelley LA, Mezulis S, Yates CM, Wass MN, Sternberg MJE. The Phyre2 web portal for protein modeling, prediction and analysis. Nat Protoc. 2015; 10:845-858. doi:10.1038/nprot.2015.053
41. Zheng W, Zhang C, Li Y, Pearce R, Bell EW, Zhang Y. Folding non-homologous proteins by coupling deep-learning contact maps with I-TASSER assembly simulations. Cell Rep Meth. 2021; 1(3):100014. doi:10.1016/j.crmeth.2021.100014
42. Leaver-Fay A, Tyka M, Lewis SM, Lange OF, Thompson J, Jacak R, et al. Rosetta3: An object-oriented software suite for the simulation and design of macromolecules. Methods Enzymol. 2011; 487:545-574. doi:10.1016/B978-0-12-381270-4.00019-6
43. Wardah W, Khan MGM, Sharma A, Rashid MA. Protein secondary structure prediction using neural networks and deep learning: A review. Comput Biol Chem. 2019; 81:1-8. doi:10.1016/j.compbiolchem.2019.107093
44. Torrisi M, Pollastri G, Le Q. Deep learning methods in protein structure prediction. Comput Struct Biotech J. 2020; 18:1301-1310. doi:10.1016/j.csbj.2019.12.011
45. Kuhlman B, Bradley P. Advances in protein structure prediction and design. Mol Cell Biol. 20(11):681-697. doi:10.1038/s41580-019-0163-x
46. Tunyasuvunakool K, Adler J, Wu Z, Green T, Zielinski M, Židek A, et al. Highly accurate protein structure prediction for the human proteome. Nature. 2021; 596(7873):590-596. doi:10.1038/s41586-021-03828-1
47. Senior AW, Evans R, Jumper J, Kirkpatrick J, Sifre L, Green T, et al. AlphaFold: Improved protein structure prediction using potentials from deep learning. Nature. 2020; 577(7792):706-710. doi:10.1038/s41586-019-1923-7
48. Maveyraud L, Mourey L. Protein X-ray crystallography and drug discovery. Molecules. 2020; 25(5):1030. doi:10.3390/molecules25051030
49. Sugiki T, Furuita K, Fujiwara T, Kojima C. Current NMR techniques for structure-based drug discovery. Molecules. 23(148):1-27. doi:10.3390/molecules23010148
50. Batool M, Ahmad B, Choi S. A structure-based drug discovery paradigm. Int J Mol Sci. 2019; 20(11):2783. doi:10.3390/ijms20112783
51. Scapin G. Structural biology and drug discovery. Curr Pharm Design. 2006; 12(17):2087-2097. doi:10.2174/138161206777585201
52. Nero TL, Parker MW, Morton CJ. Protein structure and computational drug discovery. Biochem Soc T. 2018; 46(5):1367-1379. doi:10.1042/BST20180202
53. Erlanson DA, Davis BJ, Jahnke W. Fragment-based drug discovery: Advancing fragments in the absence of crystal structures. Cell Chem Biol. 2019; 26(1):9-15. doi:10.1016/j.chembiol.2018.10.001
54. Renaud J-P, Chari A, Ciferri C, Liu W-T, Rémigy H-W, Stark H, et al. Cryo-EM in drug discovery: Achievements, limitations and prospects. Nat Rev Drug Discov. 2018; 17:471-492. doi:10.1038/nrd.2018.77
55. Saur M, Hartshorn MJ, Dong J, Reeks J, Bunkoczi G, Jhoti H, et al., Fragment-based drug discovery using cryo-EM. Drug Discov Today. 2020; 25(3):485-490. doi:10.1016/j.drudis.2019.12.006
56. Shepherd DC, Dalvi S, Ghosal D. From cells to atoms: Cryo-EM as an essential tool to investigate pathogen biology, host-pathogen interaction, and drug discovery. Mol Microbiol. 2022; 117:610-617. doi:10.1111/mmi.14820
57. Sen R, Nayak L, De RK. A review on host-pathogen interactions: Classification and prediction. Eur J Clin Microbiol Infect Dis. 2016; 35:1581-1599. doi:10.1007/s10096-016-2716-7
58. Mariano R, Wuchty S. Structure-based prediction of host-pathogen protein interactions. Curr Op Struct Biol. 2017; 44:119-124. doi:10.1016/j.sbi.2017.02.007
59. Iadanza MG, Jackson MP, Hewitt EW. A new era for understanding amyloid structures and disease. Nat Rev Mol Cell Biol. 2018; 19(12):755-773. doi:10.1038/s41580-018-0060-8
60. Willbold D, Strodel B, Schröder GF, Hoyer W, Heise H. Amyloid-type protein aggregation and prion-like properties of amyloids. Chem Rev. 2021; 121:8285-8307. doi:10.1021/acs.chemrev.1c00196
61. Hampel H, Hardy J, Blennow K, Chen C, Perry G, Kim SH, et al. The amyloid-β pathway in Alzheimer’s disease. Mol Psychiatr. 2021; 26:5481-5503. doi:10.1038/s41380-021-01249-0
62. Soto C, Pritzkow S. Protein misfolding, aggregation and conformational strains in neurodegenerative diseases. Nat Neurosci. 2018; 21(10):1332-1340. doi:10.1038/s41593-018-0235-9
63. Chen G-F, Xu T-H, Yan Y, Zhou Y-R, Jiang Y, Melcher K, Xu HE. Amyloid beta: Structure, biology and structure-based therapeutic development. Acta Pharm Sinic. 2017; 38:1205-1235. doi:10.1038/aps.2017.28
64. Zaman M, Khan AN, Wahiduzzaman, Zakariya SM, Khan RH. Protein misfolding, aggregation and mechanism of amyloid cytotoxicity: An overview and therapeutic strategies to inhibit aggregation. Int J Biol Macromol. 2019; 134:1022-1037. doi:10.1016/j.iijbiomac.2019.05.109
65. Neira JL. Fluorescence, circular dichroism and mass spectrometry as tools to study virus structure. In: Mateu M, ed. Structure and Physics of Viruses. Subcellular Biochemistry, vol.68. Dordrecht, Springer. 2013: 177-202. doi:10.1007/978-94-007-6552-8_6
66. Shanmugam G, Polavarapu PL, Kendall A, Stubbs G. Structures of plant viruses from vibrational circular dichroism. J Gen Virol. 2005; 86:2371-2377. doi:10.1099/vir.0.81055-0
67. Ksenofontov AL, Petoukhov MV, Prusov AN, Fedorova NV, Shtykova EV. Characterization of tobacco mosaic virus virions and repolymerized coat protein aggregates in solution by small-angle X-ray scattering. Biochem (Mosc.). 2020; 85(3):310-317. doi:10.1134/S0006297920030062
68. Uetrecht C, Heck AJR. Modern biomolecular mass spectrometry and its role in studying virus structure, dynamics, and assembly. Angew Chem Int Ed. 2011; 50:8248-8262. doi:10.1002/anie.201008120
69. Lecoq L, Fogeron M-L, Meier BH, Nassal M, Böckmann A. Solid-state NMR for studying the structure and dynamics of viral assemblies. Viruses. 2020; 12:1069. doi:10.3390/v12101069
70. Porat-Dahlerbruch G, Goldbourt A, Polenova T. Virus structures and dynamics by magic-angle spinning NMR. Annu Rev Virol. 2021; 8:219-237. doi:10.1146/annurev-virology-011921-064653
71. Klug A. From virus structure to chromatin: X-ray diffraction to three-dimensional electron microscopy. Annu Rev Biochem. 2010; 79:1-35. doi:10.1146/annurev.biochem.79.091407.093947
72. Luque D, Castón JR. Cryo-electron microscopy for the study of virus assembly. Nat Chem Biol. 2020; 16(3):231-239. doi:10.1038/s41589-020-0477-1
73. Prasad BVV, Schmid MF. Principles of virus structural organization. In: Rossmann M, Rao V. eds. Viral Molecular Machines. Advances in Experimental Medicine and Biology. Vol 276. Boston, Springer. 2012:17-47. doi:10.1007/978-1-4614-0980-9_3
74. Johnson JE, Chiu W. Structures of virus and virus-like particles. Curr Opin Struc Biol. 2000; 10:229-235. doi:10.1016/S0959-440X(00)00073-7
75. Plavec Z, Pöhner I, Poso A, Butcher SJ. Virus structure and structure-based antivirals. Curr Opin Virol. 2021; 51:16-24. doi:10.1016/j.coviro.2021.09.005
76. Ballester PJ. Machine learning for molecular modelling in drug design. Biomolecules. 2019; 9(6):216. doi:10.3390/biom9060216
77. Barril X, Soliva R. Molecular modelling. Mol Biosyst. 2006; 2(12):660-681. doi:10.1039/B613461K
78. Forster MJ. Molecular modelling in structural biology. Micron. 2002; 33(4):365-384. doi:10.1016/S0968-4328(01)00035-X
79. Genheden S, Reymer A, Saenz-Méndez P, Eriksson LA. Chapter 1: Computational chemistry and molecular modelling basics. In: Martín-Santamaría S, ed. Computational Tools for Chemical Biology. The Royal Society of Chemistry. 2018: 1-38. doi:10.1039/9781788010139-00001
80. Berman HM, Westbrook J, Feng Z, Gilliland G, Bhat TN, Weissig H, et al. The protein data bank. Nuc Ac Res. 2000; 28(1):235-242. doi:10.1093/nar/28.1.235
81. Berman HM, Olson WK, Beveridge DL, Westbrook J, Gelbin A, Demeny T, et al. The nucleic acid database. A comprehensive relational database of three-dimensional structures of nucleic acids. Biophys J. 1992; 63(3):751. doi:10.1016/S0006-3495(92)81649-1
82. Egorova KS, Toukach PV. Chapter 5. Carbohydrate structural database (CSDB): Examples of usage. In: Aoki-Kinoshita KF, ed. A Practical Guide to Using Glycomics Databases. Tokyo, Springer. 2017:75-113. doi:10.1007/978-4-431-56454-6_5