Understanding Single Cell Sequencing, How It Works and Its Applications
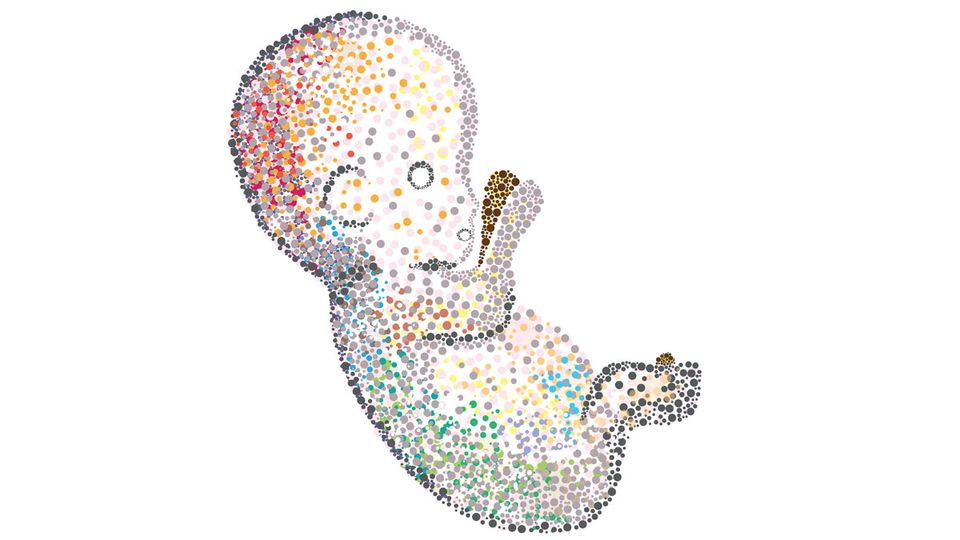
Complete the form below to unlock access to ALL audio articles.
Sequencing a nucleic acid molecule, such as DNA or RNA, yields information on the order of its nucleotides (adenine, thymine, cytosine, and guanine), which provide the genetic instructions required for life. The development of sequencing technologies stemmed from the pioneering works of Walter Fiers, Frederick Sanger and Ray Wu in the 1970s.1 In a few decades, the methods and technologies first used to sequence nucleic acids went through a steep development: from reading a single RNA molecule, it became possible to sequence the genome of an entire organism.2 The first human genome draft was published, within the Human Genome Project, in 20013 and completed two years later.
What is single cell sequencing and what is single cell RNA sequencing?
How does single cell sequencing work?
- Isolation of cells and basic sample processing
- Sequencing library preparation
- Sequencing
Types of single cell sequencing
- Single cell genome sequencing
- Single cell transcriptome sequencing (single cell RNA sequencing, single cell RNA-seq, or scRNA seq)
- Single cell DNA methylome sequencing
Analyzing single cell sequencing data
Why is single cell sequencing important?
Combining single cell sequencing with other techniques and single cell technology
Commercial DNA sequencers were first produced in the late 1990s. They have been named second-generation or next-generation sequencers to distinguish them from the first experimental ones, and allowed the sequencing of entire genomes. During the next two decades, sequencers went through rapid evolution, optimizing, to different extents, parameters such as speed, accuracy, sequencing depth and read length.4 A variety of sequencing methods have been developed for specific applications. While relatively recent, one of the most interesting ones is single cell sequencing. This article explores how this technology works and what it tells us.
What is single cell sequencing and what is single cell RNA sequencing?
Traditional next-generation sequencing (NGS) examines the genome of a cell population, such as a cell culture, a tissue, an organ or an entire organism. Its output is the “average genome” of the cell population. On the other hand, single cell sequencing measures the genomes of individual cells from a cell population.5 Nowadays, traditional methods are thus referred to as bulk sequencing to distinguish them from single cell techniques.
Single cell sequencing technologies can currently be used to measure the genome (scDNA-seq), the DNA-methylome or the transcriptome (scRNA-seq) of each cell of a population. These technologies have been used to identify novel mutations in cancerous cells, explore the progressive epigenome variations occurring during embryonic development and assess how a seemingly homogeneous cells’ population expresses specific genes (Figure 1).6
Figure 1: Using single cell sequencing technologies, we can identify novel subpopulations or cellular states within a seemingly homogeneous cellular population. A) shows different ways in which a cell population may exhibit heterogenicity. B) shows how cell types within populations may be identified and characterized.
How does single cell sequencing work?
All single cell sequencing technologies require four main steps (Figure 2):
1) Isolation of single cells from a cell population.
2) Extraction, processing and amplification of the genetic material of each isolated cell.
3) Preparation of a “sequencing library” including the genetic material of an isolated cell.
4) Sequencing of the library using a next-generation sequencer.
Figure 2: Single cell sequencing workflow. Depending on the genetic material of interest, the sample preparation procedure differs slightly. In all cases, however, the resulting sequencing material is a library of barcoded DNA or cDNA for each cell which can then be sequenced on the chosen sequencing platform.
Isolation of cells and basic sample processing
Cells can be isolated using different methods,7,8 the choice of which mainly depends on the nature of the sample and the processing steps required after the cells’ isolation. The performance of each method is defined by its efficiency (how many cells can be isolated per unit of time), purity (the fraction of target cells collected) and recovery (the fraction of target cells collected compared to the total number of target cells initially available). Let us consider the most commonly used techniques.9
- Fluorescence-activated cell sorting (FACS) involves labeling the cell proteins on which cell selection is based with fluorescent molecules attached to target-specific antibodies. Intracellular markers can be accessed using cell permeabilization. The technique thus allows cells to be selected based on multiple parameters. However, FACS requires >10,000 starting cells, and the fast flow may damage the cells’ viability.7
- Magnetic-activated cell sorting (MACS) uses antibody-mediated superparamagnetic nanoparticles to tag specific proteins on the target cells. However, this means that, unlike with FACS, only cell surface molecules can be used as a target to tag live cells.10 An external magnetic field is then used to isolate the tagged cells while the others are washed away. Therefore, the purity of a MACS isolation depends on the specificity and affinity of the antibodies used for tagging.
- Laser capture microdissection (LCM) uses a laser to isolate target cells from a solid tissue sample placed on a microscope slide. The isolation can be done in two ways: directly, when infrared light transfers the laser energy to a thermolabile polymer that specifically binds target cells only; indirectly, when ultraviolet light ablates the cell. Unlike FACS and MACS, LCM can be used on intact tissues. It is also rapid and reliable. However, LCM requires the identification of the target cells through visual inspection of their morphology. Also, cells may be sliced during isolation, and ultraviolet light may damage the DNA and RNA molecules.11
- Finally, manual cell picking, or micromanipulation, requires an inverted microscope combined with micro-pipettes for selecting and isolating the target cells. Micromanipulation has been used with live cultures and embryonic cells. However, its throughput is limited and, as with LCM, the technique requires skilled professionals to identify the target cells correctly.12
The quality of the cell isolation is evaluated before proceeding with the library preparation, and the viability of the cells is assessed by imaging. RNA integrity can also be evaluated, particularly important for scRNA-seq analyses. The isolated cells are then lysed. The genetic material of interest (DNA or RNA) is isolated and amplified to provide enough for subsequent detection as single cells typically yield only tiny quantities of DNA or RNA. The resulting material of these steps is single-stranded DNA, even for scRNA-seq analyses, as explained in the next section. A number of protocols have been developed to deal with the requirements and limitations of specific studies, such as small numbers of available cells.13
Resolve the Complex Cellular Diversity of Your Samples

Download this app note to discover a custom service that provides access to two multiomic platforms allowing you easy and cost-effective gene expression profiling of up to tens of thousands of cells, convenient, powerful data analysis and visualization and full expert guidance on your single-cell RNA-Seq project.
View App NoteSponsored Content
Resolve the Complex Cellular Diversity of Your Samples

Download this app note to discover a custom service that provides access to two multiomic platforms allowing you easy and cost-effective gene expression profiling of up to tens of thousands of cells, convenient, powerful data analysis and visualization and full expert guidance on your single-cell RNA-Seq project.
View App NoteSponsored Content
Sequencing library preparation
For the amplified DNA to be sequenced, it first needs to be made into a sequencing library.13,14 A sequencing library is a collection of single-stranded DNA fragments derived from one cell population or, in the case of single cell sequencing, from one specific cell. After amplification, the DNA fragments are uniquely barcoded to identify which starting cell they belong to, and specific adapter sequences are added to the 5’ and 3’ ends. At this point, the DNA portion that needs to be sequenced is usually named insert. The barcodes and adapters cap the insert at one or both ends. All the DNA fragments belonging to the same sequencing library are barcoded using the same oligonucleotide sequences. This allows the pooling of different libraries to be sequenced together during the same sequencing run. The adapters are platform-dependent and are needed to sequence the fragments. Commercial kits are available for all the sample and library preparation steps. To ensure that the resulting library accurately reproduces the original cell’s state, several quality controls have been described (Figure 3).15
Figure 3: Quality controls for evaluating library preparation and sequencing outcomes. In this example, single cells have been isolated by FACS.
Sequencing
The various commercially available sequencing platforms have developed slightly different methods. Here, we focus on the sequencing by synthesis method, including variations such as pyrosequencing and reversible terminator sequencing. Before sequencing, an amplification step usually generates groups of DNA fragment clones (typically by bridge amplification or emulsion PCR). As each set of clones emits identical signals during sequencing, the resulting cluster or well signals are strong enough for detection.16This type of sequencing usually takes place within a chip, which may contain micro-wells. Adapters and other molecules, like polymerases, are bound to the chip (or to the bottoms of the micro-wells) and interact with the adapters attached to the inserts. The insert sequencing then requires multiple replication steps, performed by the polymerases and using fluorescently tagged nucleotides. During each cycle, a single fluorescently labeled nucleotide is added and, if incorporated by the polymerase, light emission is triggered that is characteristic for the specific nucleotide. The light spectra simultaneously emitted by all the fragments are recorded through a camera before the next cycle begins. As each nucleotide emits a different light, the sequencer reconstructs, cycle by cycle, the sequence of all the inserts. The sequencer also reads the inserts’ tags to assign each measurement to its appropriate library.
Proton detection sequencing uses a different sequencing by synthesis method. Fragments are usually bound to beads and amplified to cover each bead (similar to pyrosequencing). However, when bases are added during the sequencing process, instead of a fluorescent tag and light release, it releases a single proton which can then be detected and recorded.
Another less common sequencing method is sequencing by ligation. This method uses DNA ligases instead of DNA polymerases, which attach fluorescently tagged short sequences instead of nucleotides. Before sequencing, DNA fragments are usually amplified using emulsion PCR amplification chemistry. As each nucleotide of the insert is then sequenced twice, this method provides very accurate reads. However, sequencing by ligation outputs shorter reads only and is incompatible with palindromic sequences.
Types of single cell sequencing
Single cell sequencing technologies can measure different types of genetic material – the genome, the transcriptome or the methylome – of a single cell. This section explains the main differences in sample preparation (Figure 2) and the most relevant applications of these three sequencing subtypes.
Single cell genome sequencing
By determining the genome of single cells, scDNA-seq allows the genomic heterogeneity of a cellular population to be investigated.17 As such, it is mainly used to study microbiomes and cancer. Microbiomes are communities of unicellular organisms, and scDNA-seq measures the genomes of their microbial components without the need to isolate and cultivate them first. The sequencing data can then be used to study the microbiome’s composition and, therefore, its ecology, evolution and alterations.18,19 In cancer research, scDNA-seq is used to study intra-tumor genetic heterogeneity or to identify novel cancerogenic mutations.20,21 Due to these innovative capacities, scDNA-seq significantly boosted the development of precision medicine.22
For scDNA-seq, the DNA extracted from isolated cells is most frequently amplified using multiple displacement amplification (MDA) or multiple annealing and looping-based amplification cycles (MALBAC).23 MDA allows the fast amplification of minute amounts of DNA, providing an excellent but uneven genome coverage. MALBAC, on the other hand, provides a reduced but more even coverage of the genome and is thus better suited for detecting copy number variants. Libraries are generated, as previously described, from the amplified DNA. For this method to succeed, an even and efficient amplification is crucial. However, no amplification method is faultless. In particular, detecting single-nucleotide polymorphisms (SNPs) and copy number variations is often highly inefficient. Different amplification methods have thus been developed to improve the detection of specific mutation types.24
Single cell transcriptome sequencing (single cell RNA sequencing, single cell RNA-seq, or scRNA seq)
scRNA-seq measures the RNA molecules within each cell of a given sample. This information provides a snapshot of the transcriptome (the genes that were being transcribed) when the cells were harvested.25,26 Since its development, scRNA-seq has found a large variety of applications. Although the final product of a gene’s expression is a protein, detecting its messenger RNA (mRNA) indicates the gene is turned on and, therefore, has the potential to be subsequently translated and expressed. Furthermore, co-transcribed genes can be used to infer the gene-regulatory networks that underlie specific cells’ phenotypes.27 The transcriptional differences of a cellular population can help to identify subpopulations, such as the malignant cells of a tumor mass.28 scRNA-seq is also used to study important gene transcription characteristics, such as splicing patterns and monoallelic gene transcription.29,30 Prokaryote scRNA-seq has been problematic due to factors such as the low mRNA copy numbers in bacterial cells and RNA instability. However, methods are becoming available that overcome these issues.31,32
Isolated cells are lysed, and their mRNA molecules, which are polyadenylated, are enriched using poly[T]-primers. This is a crucial step as most RNA molecules in a cell are ribosomal (rRNA): very large and, usually, not the target of transcriptome sequencing. Next, reverse transcriptases convert the poly[T]-primed, single-stranded mRNAs into complementary DNA (cDNA). PCR or in vitro transcription (IVT) are then used to amplify the cDNA molecules. Finally, barcoding tags and the other short sequences required by the sequencing platform are added to the cDNA molecules.26
The sequencing quality of this technology is affected by a few factors, the main ones being the total number of libraries that can be derived from a cell population and the detected reads. The ideal number of cells depends on the expected number of different cellular subpopulations or states. The number of reads indicates how deeply a transcriptome has been sequenced, depending on the size of the genome: a higher read depth provides more reliable details. The sample and library preparation protocol also affects the result’s quality.
Single cell DNA methylome sequencing
DNA methylation involves the transfer of a methyl group onto a cytosine carbon (often C5). Methylation is an epigenetic mechanism that changes the DNA activity without affecting its sequence: when in a gene promoter, DNA methylation usually represses the gene’s transcription.33 Single cell DNA methylome sequencing (scDNA-Met-seq) can thus be used to study the epigenetic changes within an otherwise genetically identical cellular population, giving rise to different phenotypes. DNA methylation is also essential to cellular identity and is key to X-chromosome inactivation, genomic imprinting, repression of transposable elements, aging and carcinogenesis. This technology is mainly used in developmental studies,34 but has also been employed to explore rare and extremely active subpopulations of tumor cells.35
The samples and library preparations for scDNA-Met-seq are similar to those for scDNA-seq with an additional step. Prior to amplification, the DNA undergoes bisulfite conversion, which only converts the non-methylated cytosine residues into uracil, leaving 5-methylcytosine residues unaffected.36 However, the bisulfite treatment tends to fragment and degrade the DNA. Other methods, such as methylation-sensitive restriction enzymes, have been explored but are still unavailable for single cell sequencing.
Analyzing single cell sequencing data
The final raw output of the sequencer is first processed directly within the sequencing machine, returning the binary base call (BCL) file and quality scores. The BCL file is the raw sequencing output in binary format. For further analysis, the BCL file is then converted into a FASTQ file, a text file containing the sequence information and quality scores (Figure 3). This step is usually performed on a Linux server after demultiplexing the data from different libraries using the barcode tags.
The FASTQ files can be processed to align the sequences to a template genome, annotate them, detect variants, perform differential transcription analyses and visualize the data.37,38 Several third-party academic-developed scripts are available to perform these preliminary analyses.39,40 Given the size of the FASTQ data files (typically 10-200 gigabytes each), most of these analyses are computationally expensive and, thus, usually performed on a Linux server using its command line. The resulting data can be investigated further using data analysis and statistical tools, such as data normalization, principal component analysis, t-distributed stochastic neighbor embedding analysis, cluster analysis and pathway or gene set enrichment analyses. These tools are helpful when exploring large datasets as they allow unexpected patterns and biological behaviors to be identified, as well as the genes or transcripts that most significantly drive specific phenotypes. In particular, Bioconductor is a sundry package developed for the R statistical programming language, providing free, open-source software for the analysis of genomics data.41 The tools within this package have been designed to perform the above-mentioned analyses and visualize their results. Certain workflows and functions have been specifically optimized for single cell sequencing analyses.42,43
Why is single cell sequencing important?
Each cell within a tissue or organ contributes, in different ways, to the physio/pathology of the entire organism. With single cell technologies, we can probe each cell and measure its specific contribution to the whole cell population – and its organism or ecosystem. This unique level of detail is particularly valuable when studying rare cells or exploring the phenotype variations within same-cell-type populations. For instance, scRNA-seq has been used to investigate rare antigen-specific T or B cells,44 measure the composition and structure of human microbiomes,45 study the origin and development of chemoresistant tumor subpopulations,46 discover previously unknown genes’ functions in plant tissues,47 study tumor progression mechanisms and base prognostic predictions on intra-tumor cellular heterogeneity.48 These and many more applications have been made possible in various fields by the uniqueness of single cell sequencing technologies.
Combining single cell sequencing with other techniques and single cell technology
Various omics technologies are now frequently combined to investigate the multilayered state of single cells.49,50 By combining the previously described sequencing technologies, it is possible to study the genomic, epigenomic and transcriptomic landscapes within the same cellular population.51,52 The sequencing technologies are also frequently combined with proteomics approaches, both bulk and single cell, including metabolomics, phosphoproteomics, acetylomics and glycoproteomics.53,54 Combining different single cell omics methods makes it possible to reach a deeper understanding of a cellular population’s heterogeneity: more subpopulations may be identified, as other technologies may pick up different types of variations. It may also be possible to infer functional connections between the alterations observed by one omics technique with those observed by another. This information could help to identify new cause-effect relationships and, thus, the mechanisms behind a known phenotype.
Several computational methods have been developed to integrate different omics datasets,55,56 including innovative, machine learning-based approaches.57 However, the algorithms integrating multi-omics single cell data are still often inadequate. While library preparation protocols and sequencing technologies have been greatly perfected, data analysis tools have lagged behind and now possibly pose the greatest challenge of this field.
References
1. Jou WM, Haegeman G, Ysebaert M, Fiers W. Nucleotide sequence of the gene coding for the bacteriophage MS2 coat protein. Nature. 1972;237(5350):82-88. doi:10.1038/237082a0
2. Sanger F, Air GM, Barrell BG, et al. Nucleotide sequence of bacteriophage φX174 DNA. Nature. 1977;265(5596):687-695. doi:10.1038/265687a0
3. Venter JC, Adams MD, Myers EW, et al. The sequence of the human genome. Science. 2001;291(5507):1304-1351. doi:10.1126/science.1058040
4. Liu L, Li Y, Li S, et al. Comparison of next-generation sequencing systems. J Biomed Biotechnol. 2012;2012:1-11. doi:10.1155/2012/251364
5. Tang X, Huang Y, Lei J, Luo H, Zhu X. The single-cell sequencing: new developments and medical applications. Cell Biosci. 2019;9(1):53. doi:10.1186/s13578-019-0314-y
6. Mannarapu M, Dariya B, Bandapalli OR. Application of single-cell sequencing technologies in pancreatic cancer. Mol Cell Biochem. 2021;476(6):2429-2437. doi:10.1007/s11010-021-04095-4
7. Gross A, Schoendube J, Zimmermann S, Steeb M, Zengerle R, Koltay P. Technologies for single-cell isolation. Int J Mol Sci. 2015;16(8):16897-16919. doi:10.3390/ijms160816897
8. Valihrach L, Androvic P, Kubista M. Platforms for single-cell collection and analysis. Int J Mol Sci. 2018;19(3):807. doi:10.3390/ijms19030807
9. Hu P, Zhang W, Xin H, Deng G. Single cell isolation and analysis. Front Cell Dev Biol. 2016;4. doi:10.3389/fcell.2016.00116
10. Welzel G, Seitz D, Schuster S. Magnetic-activated cell sorting (MACS) can be used as a large-scale method for establishing zebrafish neuronal cell cultures. Sci Rep. 2015;5(1):7959. doi:10.1038/srep07959
11. Espina V, Wulfkuhle JD, Calvert VS, et al. Laser-capture microdissection. Nat Protoc. 2006;1(2):586-603. doi:10.1038/nprot.2006.85
12. Citri A, Pang ZP, Südhof TC, Wernig M, Malenka RC. Comprehensive qPCR profiling of gene expression in single neuronal cells. Nat Protoc. 2012;7(1):118-127. doi:10.1038/nprot.2011.430
13. Hess JF, Kohl TA, Kotrová M, et al. Library preparation for next generation sequencing: A review of automation strategies. Biotechnol Adv. 2020;41:107537. doi:10.1016/j.biotechadv.2020.107537
14. Syed F, Grunenwald H, Caruccio N. Next-generation sequencing library preparation: simultaneous fragmentation and tagging using in vitro transposition. Nat Methods. 2009;6(11):i-ii. doi:10.1038/nmeth.f.272
15. Endrullat C, Glökler J, Franke P, Frohme M. Standardization and quality management in next-generation sequencing. Appl Transl Genom. 2016;10:2-9. doi:10.1016/j.atg.2016.06.001
16. Pareek CS, Smoczynski R, Tretyn A. Sequencing technologies and genome sequencing. J Appl Genet. 2011;52(4):413-435. doi:10.1007/s13353-011-0057-x
17. Gawad C, Koh W, Quake SR. Single-cell genome sequencing: current state of the science. Nat Rev Genet. 2016;17(3):175-188. doi:10.1038/nrg.2015.16
18. Kashtan N, Roggensack SE, Rodrigue S, et al. Single-cell genomics reveals hundreds of coexisting subpopulations in wild prochlorococcus. Science. 2014;344(6182):416-420. doi:10.1126/science.1248575
19. Swan BK, Tupper B, Sczyrba A, et al. Prevalent genome streamlining and latitudinal divergence of planktonic bacteria in the surface ocean. PNAS. 2013;110(28):11463-11468. doi:10.1073/pnas.1304246110
20. Zhang L, Dong X, Lee M, Maslov AY, Wang T, Vijg J. Single-cell whole-genome sequencing reveals the functional landscape of somatic mutations in B lymphocytes across the human lifespan. PNAS. 2019;116(18):9014-9019. doi:10.1073/pnas.1902510116
21. Francis JM, Zhang CZ, Maire CL, et al. EGFR variant heterogeneity in glioblastoma resolved through single-nucleus sequencing. Cancer Discov. 2014;4(8):956-971. doi:10.1158/2159-8290.CD-13-0879
22. Wiedmeier JE, Noel P, Lin W, von Hoff DD, Han H. Single-cell sequencing in precision medicine. Cancer Treat Res. Published online 2019:237-252. doi:10.1007/978-3-030-16391-4_9
23. Chen M, Song P, Zou D, et al. Comparison of multiple displacement amplification (MDA) and multiple annealing and looping-based amplification cycles (MALBAC) in single-cell sequencing. PLoS ONE. 2014;9(12):e114520. doi:10.1371/journal.pone.0114520
24. Treff NR, Su J, Tao X, Northrop LE, Scott RT. Single-cell whole-genome amplification technique impacts the accuracy of SNP microarray-based genotyping and copy number analyses. Basic Sci Repro Med. 2011;17(6):335-343. doi:10.1093/molehr/gaq103
25. Kolodziejczyk AA, Kim JK, Svensson V, Marioni JC, Teichmann SA. The technology and biology of single-cell RNA sequencing. Mol Cell. 2015;58(4):610-620. doi:10.1016/j.molcel.2015.04.005
26. Haque A, Engel J, Teichmann SA, Lönnberg T. A practical guide to single-cell RNA-sequencing for biomedical research and clinical applications. Genome Med. 2017;9(1):75. doi:10.1186/s13073-017-0467-4
27. Wagner A, Regev A, Yosef N. Revealing the vectors of cellular identity with single-cell genomics. Nat Biotechnol. 2016;34(11):1145-1160. doi:10.1038/nbt.3711
28. Tirosh I, Izar B, Prakadan SM, et al. Dissecting the multicellular ecosystem of metastatic melanoma by single-cell RNA-seq. Science. 2016;352(6282):189-196. doi:10.1126/science.aad0501
29. Shalek AK, Satija R, Adiconis X, et al. Single-cell transcriptomics reveals bimodality in expression and splicing in immune cells. Nature. 2013;498(7453):236-240. doi:10.1038/nature12172
30. Deng Q, Ramsköld D, Reinius B, Sandberg R. Single-cell RNA-seq reveals dynamic, random monoallelic gene expression in mammalian cells. Science. 2014;343(6167):193-196. doi:10.1126/science.1245316
31. Imdahl F, Vafadarnejad E, Homberger C, Saliba A-E, Vogel J. Single-cell RNA-sequencing reports growth-condition-specific global transcriptomes of individual bacteria. Nat Microbiol. 2020;5(10):1202-1206. doi:10.1038/s41564-020-0774-1
32. Kuchina A, Brettner LM, Paleologu L, et al. Microbial single-cell RNA sequencing by split-pool barcoding. Science. 2021;371(6531):eaba5257. doi:10.1126/science.aba5257
33. Moore LD, Le T, Fan G. DNA methylation and its basic function. Neuropsychopharmacology. 2013;38(1):23-38. doi:10.1038/npp.2012.112
34. Zhu P, Guo H, Ren Y, et al. Single-cell DNA methylome sequencing of human preimplantation embryos. Nat Genet. 2018;50(1):12-19. doi:10.1038/s41588-017-0007-6
35. Gkountela S, Castro-Giner F, Szczerba BM, et al. Circulating tumor cell clustering shapes DNA methylation to enable metastasis seeding. Cell. 2019;176(1-2):98-112.e14. doi:10.1016/j.cell.2018.11.046
36. Smallwood SA, Lee HJ, Angermueller C, et al. Single-cell genome-wide bisulfite sequencing for assessing epigenetic heterogeneity. Nat Methods. 2014;11(8):817-820. doi:10.1038/nmeth.3035
37. Dobin A, Davis CA, Schlesinger F, et al. STAR: ultrafast universal RNA-seq aligner. Bioinformatics. 2013;29(1):15-21. doi:10.1093/bioinformatics/bts635
38. Trapnell C, Roberts A, Goff L, et al. Differential gene and transcript expression analysis of RNA-seq experiments with TopHat and Cufflinks. Nat Protoc. 2012;7(3):562-578. doi:10.1038/nprot.2012.016
39. Bray NL, Pimentel H, Melsted P, Pachter L. Near-optimal probabilistic RNA-seq quantification. Nat Biotechnol. 2016;34(5):525-527. doi:10.1038/nbt.3519
40. Bankevich A, Nurk S, Antipov D, et al. SPAdes: A new genome assembly algorithm and its applications to single-cell sequencing. J Comput Biol. 2012;19(5):455-477. doi:10.1089/cmb.2012.0021
41. Lawrence M, Huber W, Pagès H, et al. Software for computing and annotating genomic ranges. PLoS Comput Biol. 2013;9(8):e1003118. doi:10.1371/journal.pcbi.1003118
42. Amezquita RA, Lun ATL, Becht E, et al. Orchestrating single-cell analysis with Bioconductor. Nat Methods. 2020;17(2):137-145. doi:10.1038/s41592-019-0654-x
43. Perraudeau F, Risso D, Street K, Purdom E, Dudoit S. Bioconductor workflow for single-cell RNA sequencing: Normalization, dimensionality reduction, clustering, and lineage inference. F1000Research. 2017;6:1158. doi:10.12688/f1000research.12122.1
44. Shalek AK, Satija R, Adiconis X, et al. Single-cell transcriptomics reveals bimodality in expression and splicing in immune cells. Nature. 2013;498(7453):236-240. doi:10.1038/nature12172
45. Balachandran M, Cross KL, Podar M. Single-cell genomics and the oral microbiome. J Dent Res. 2020;99(6):613-620. doi:10.1177/0022034520907380
46. Kim C, Gao R, Sei E, et al. Chemoresistance evolution in triple-negative breast cancer delineated by single-cell sequencing. Cell. 2018;173(4):879-893.e13. doi:10.1016/j.cell.2018.03.041
47. Wendrich JR, Yang B, Vandamme N, et al. Vascular transcription factors guide plant epidermal responses to limiting phosphate conditions. Science. 2020;370(6518). doi:10.1126/science.aay4970
48. Patel AP, Tirosh I, Trombetta JJ, et al. Single-cell RNA-seq highlights intratumoral heterogeneity in primary glioblastoma. Science. 2014;344(6190):1396-1401. doi:10.1126/science.1254257
49. Lake BB, Chen S, Sos BC, et al. Integrative single-cell analysis of transcriptional and epigenetic states in the human adult brain. Nat Biotechnol. 2018;36(1):70-80. doi:10.1038/nbt.4038
50. Granja JM, Klemm S, McGinnis LM, et al. Single-cell multiomic analysis identifies regulatory programs in mixed-phenotype acute leukemia. Nat Biotechnol. 2019;37(12):1458-1465. doi:10.1038/s41587-019-0332-7
51. Kashima Y, Sakamoto Y, Kaneko K, Seki M, Suzuki Y, Suzuki A. Single-cell sequencing techniques from individual to multiomics analyses. Exp Mol Med. 2020;52(9):1419-1427. doi:10.1038/s12276-020-00499-2
52. Lee J, Hyeon DY, Hwang D. Single-cell multiomics: technologies and data analysis methods. Exp Mol Med. 2020;52(9):1428-1442. doi:10.1038/s12276-020-0420-2
53. Angelidis I, Simon LM, Fernandez IE, et al. An atlas of the aging lung mapped by single cell transcriptomics and deep tissue proteomics. Nat Commun. 2019;10(1):963. doi:10.1038/s41467-019-08831-9
54. Zhu Y, Scheibinger M, Ellwanger DC, et al. Single-cell proteomics reveals changes in expression during hair-cell development. eLife. 2019;8. doi:10.7554/eLife.50777
55. Stuart T, Butler A, Hoffman P, et al. Comprehensive integration of single-cell data. Cell. 2019;177(7):1888-1902.e21. doi:10.1016/j.cell.2019.05.031
56. Welch JD, Kozareva V, Ferreira A, Vanderburg C, Martin C, Macosko EZ. Single-cell multi-omic integration compares and contrasts features of brain cell identity. Cell. 2019;177(7):1873-1887.e17. doi:10.1016/j.cell.2019.05.006 57. Erfanian N, Heydari AA, Iañez P, et al. Deep learning applications in single-cell omics data analysis. bioRxiv. Published online January 1, 2021:2021.11.26.470166. doi:10.1101/2021.11.26.470166