Towards Improved Data Reproducibility
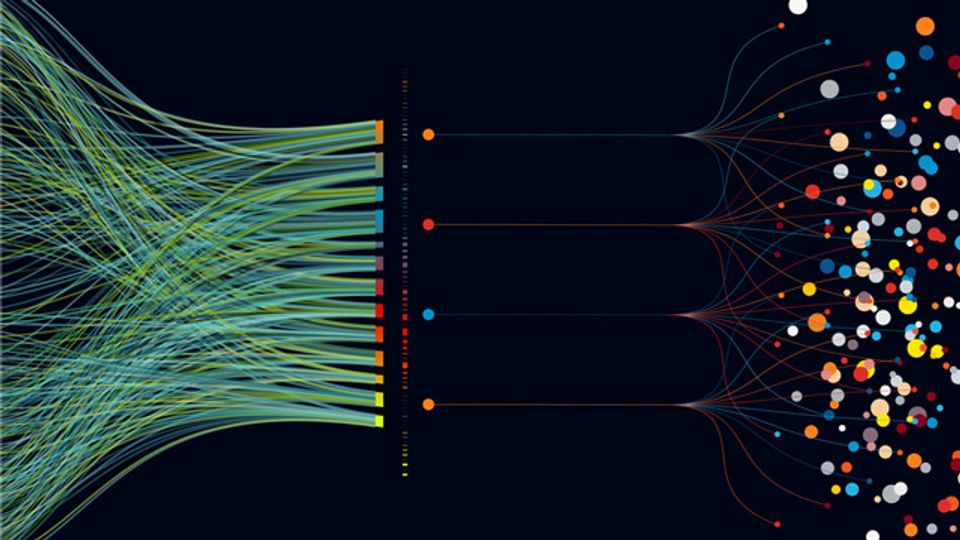
Complete the form below to unlock access to ALL audio articles.
The ability to reproduce another researcher’s work is a cornerstone of science, and yet in many cases scientists do not have access to the data or methods used by others to be able to validate and confidently build on their findings. Why is data reproducibility often so poor? And what can be done about it? In this article, we look at some of the measures we can take as individual scientists and as a global community to improve the reproducibility and rigor of scientific endeavor.
Why is data reproducibility important?
Data reproducibility is the ability to regenerate the results of an experiment, such as the data presented in a scientific publication, by using the authors’ datasets, analysis methods and codes. It is distinct from replicability, which is repeating an experiment using a different setup and arriving at the same results. Both are an important part of robust scientific research for a range of different reasons.
“Reproducibility is the foundation of every scientific field,” says Susanna-Assunta Sansone, associate professor in data readiness at the University of Oxford and associate director of the Oxford e-Research Centre. “Science is about continuity: discoveries are made using other people’s data, and advancements are made using our collective knowledge. However, data and knowledge that cannot be reproduced, verified and therefore trusted helps no one. It lacks any potential and is of no use.”
In addition to being able to trust the foundations of scientific knowledge, there are also more selfish reasons for taking data reproducibility seriously. Florian Markowetz is a senior group leader at the Cancer Research UK Cambridge Institute and a local network lead for the UK Reproducibility Network. In his article, “Five Selfish Reasons to Work Reproducibly”,1 he argues that data reproducibility has several benefits beyond protecting the foundation of science – including avoiding data analysis disasters, making it easier to write papers and building your professional reputation.
“I have a very simple approach to data reproducibility,” Markowetz says, “If you want to have a good career, and if you want to do good science, there are certain requirements, the environment has to be set up in a certain way. For example, you have to understand how others conducted their research to really engage with them, to engage with their results. And if they did not share their data and methods with me, I will never be able to understand what they did. That for me is the problem.”
Five Essentials for Surviving Your Next Laboratory Inspection
1635874611797.png)
Even with robust quality systems governing your analytical laboratory and a strong data integrity (DI) program in place, you may still be nervous about preparing for a regulatory inspection. What will the inspectors want to know? What will they ask? Is your team prepared? Watch this webinar to discover what to expect and gain insights into how DI plays a role in the inspection and how to understand and answer the inspector’s questions.
Watch WebinarSponsored Content
In recent years, there has been growing concerns over the reproducibility of data for many reasons, with reports of a “reproducibility crisis” in some fields.2 So why is data reproducibility a growing concern?
“First, the growing scale and diversity of the scholarly digital asset (including datasets, code, models, article, preprints, protocols) is placing strain on the mechanisms we currently have for peer review and quality control of the information that is shared,” says Sansone. “Second, a vast majority of datasets and code that is in the public domain is still not reusable, for a number of reasons. Even if it is openly available, it is very often poorly described, which means it is not suitable for third party use.”
A considerable barrier to this is that datasets still require a substantial amount of preparation before researchers can begin to re-use them to answer research questions. Preparing data for sharing takes time and effort.
“One thing that’s important to recognize is that in the short-term, the steps you need to take to make data reproducible are not making your life easier, because you have to learn all kinds of new tools,” says Markowetz. “If you just want to hack together a table with all the information you have, I’m pretty sure that Excel is quicker than learning how to program and tidy up your data. So really, you have to view it as a long-term investment.”
In Markowetz’ lab, they approach reproducibility by using data management and versioning tools available in Python, R and Github at different stages of collection and analysis. “A general problem is managing messy data, where there may be ten or more different spellings of a scientific term or drug name, and somebody has to clean this up – this is an important step towards tidy data,” he explains. “Then once we know which direction we want to take with our analysis, we try to document as much as we can because we hope that, if successful, this will be our next paper. And we don’t want to be in a stupid situation where we have forgotten how we got our results. The most practical step towards data reproducibility in a multidisciplinary environment is that everyone knows how to program the software language and knows how versioning systems such as GitHub work, and then you’re almost there.”
Avoid False Positive ELISA Data

Enzyme Linked Immunosorbent Assays (ELISAs) are commonly used to quantify biomarkers in serum, plasma and cell culture supernates. These samples contain a variety of factors that can interfere with ELISA results, commonly referred to as a matrix effect. Download this app note to learn more about the importance of blocking reagents when detecting Human GDNF and testing the importance of blockers in other Quantikine Elsa Kits.
View App NoteSponsored Content
System-wide efforts to improve data reproducibility
Thankfully, as more funders and publishers set expectations for more open access to data, there are more tools being developed to support this and much more guidance and training available on how to work in a reproducible way.
In 2016, together with a group of internationally recognized leaders in data management, Sansone co-authored the FAIR Principles – a set of guiding tenets to ensure that contemporary data resources and scholarly output are Findable, Accessible, Interoperable and Reusable (FAIR).3 These are already being widely adopted by many stakeholders in the scientific ecosystem, and have propelled the global debate about better data stewardship.
“Funding bodies are now consolidating FAIR principles into their funding agreements, publishers have united behind FAIR as a way to remain at the forefront of open research and in the private sector FAIR is being adopted and enshrined in policy in major biopharmas, libraries and unions,” says Sansone. “The principles have also been endorsed by global and intergovernmental leaders such as G20, the G7 Expert Group on Open Science, and the Organization for Economic Co-operation and Development (OECD) Committee for Scientific and Technological Policy, making them a de facto global norm for good research data management and a prerequisite for data science.”
But although the FAIR principles have accelerated global discussion about better data stewardship across all disciplines, they still need to be turned into practice. Although there have been some improvements, data still rarely follows the FAIR principles and they continue to be aspirational.
Leveraging Extended Reality (XR) Technology To Drive Laboratory Productivity

To maximize the output of the lab, scientists need to be able to rely on technological advancements, innovative software and improved processes. Ultimately, increasing productivity by streamlining day to day activities is a main component in becoming a lab of the future. Watch the webinar to learn more about the value of a fully connected ecosystem and how to achieve this in your laboratory.
Watch WebinarSponsored Content
Turning FAIR principles into practice
A number of communities worldwide are working to turn FAIR into reality. “This is being done by designing and implementing relevant technological and social infrastructure, as well as cultural and policy changes, supported by new educational and training elements,” says Sansone. “This work is done not just with researchers, but engaging all stakeholders involved in the data life cycle: from developers, service providers, librarians, journal publishers, funders, societies in the academic as well as in the commercial and governmental setting.”
One such example is the UKRN, which is led by Professor Marcus Munafo at Bristol University and for which Markowetz is the local lead for Cambridge. “It was set up because reproducibility is a big problem that is bigger than individual institutions and individual disciplines,” explains Markowetz. “So, it makes sense to address it with a much more coherent approach across the country, and there are lots of reproducibility networks now in different countries.” The research culture and landscape differ between countries, so we have to tackle this at a national level. It’s a UK-wide initiative to improve reproducibility across disciplines from psychology to political sciences and biomedicine.” The UKRN is tackling the issue in two ways: through high-level collaboration with funders and building infrastructure to support reproducibility, and at grassroots level through training workshops about how to conduct science reproducibly which cover everything from programming through to leadership.
“What’s really needed is for the research leaders at universities to be more explicit in their support for data reproducibility,” says Markowetz, “We have these large national efforts like the UKRN, and lots of excitement and engagement from early career researchers, but what we don’t yet have is the middle part, which is senior leadership in individual universities taking this seriously and changing their tenure process or academic career progression framework, for example.”
In Oxford, Sansone is helping to support the efforts of the UKRN and similar national initiatives in other countries though the work of her Data Readiness Group, which researches and develop methods and tools to improve data reuse. Her team has worked collaboratively with international stakeholders to produce FAIR-enabling resources such as FAIRsharing and the FAIR Cookbook, with major pharmaceutical companies supporting the work of the UKRN and its counterparts in other countries.4
Ultimately though, it will come down to individual practices in the research community. “The community needs to help itself by transforming the research culture (the environment in which we do research) and its research practices (how we do research),” says Sansone. “Science is a team sport, and teamwork is hard: but you have to play your part. Better data means better science.”
References
1. Markowetz F. Five selfish reasons to work reproducibly. Genome Biol. 2015;16:274.. doi: 10.1186/s13059-015-0850-7
2. Baker M. 1,500 scientists lift the lid on reproducibility. Nature 2016;533:452–454. doi: 10.1038/533452a
3. Wilkinson MD, Dumontier M, Aalbersberg IJ, et al. The FAIR Guiding Principles for scientific data management and stewardship [published correction appears in Sci Data. 2019 Mar 19;6(1):6]. Sci Data 2016;3:160018. doi: 10.1038/sdata.2016.18
4. Sansone SA, McQuilton P, Rocca-Serra P, et al. FAIRsharing as a community approach to standards, repositories and policies. Nat Biotechnol. 2019;37:358–367. doi: 10.1038/s41587-019-0080-8