The Binomial Test
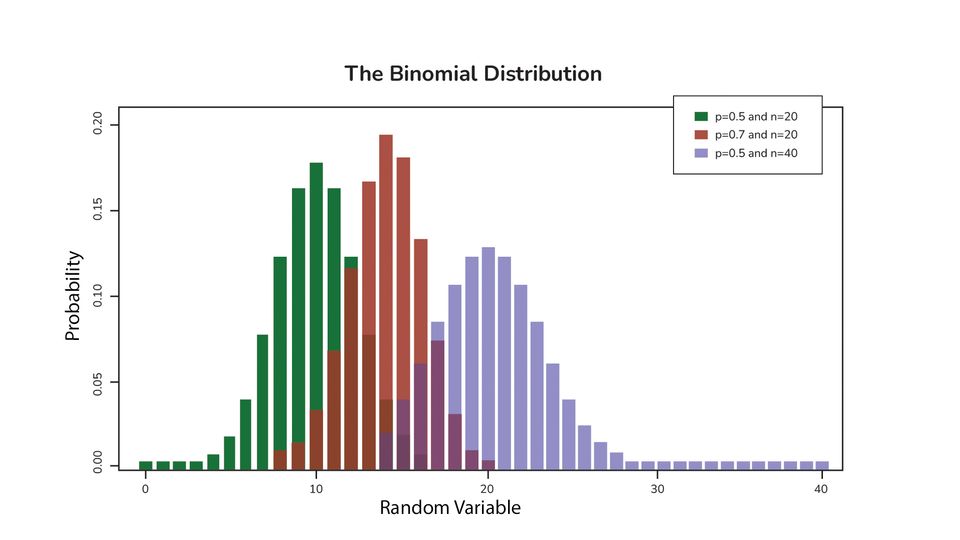
Complete the form below to unlock access to ALL audio articles.
What is the Binomial test?
The Binomial test, sometimes referred to as the Binomial exact test, is a test used in sampling statistics to assess whether a proportion of a binary variable is equal to some hypothesized value. In this article, we explore the key features of this test and walk through an example test.
What are the hypotheses of the binomial test?
The hypotheses for the Binomial test are as follows:
- The null hypothesis (H0) is that the population proportion of one outcome equals a specific hypothesized value (this can be denoted as π = πo).
- The alternative hypothesis (H1) is that the population proportion of one outcome does not equal a specific hypothesized value (π ≠ πo).
Sometimes you may also want to test a null hypothesis of the population proportion as being greater than the hypothesized value specifically (or lesser than), rather than different in any direction, in which case you would perform a one tailed significance test, but more commonly the two tailed approach is used.
Note that there is no test statistic generated in a Binomial test as is common in other statistical tests such as the Mann-Whitney U Test or the unpaired Student’s t-test, due to the p-value being calculated directly.
When to use the Binomial test
The Binomial test is used when a binary variable of interest (a variable that can take only two possible values e.g. mortality (dead/alive)) is being investigated and you have a hypothesized or expected value with which to compare it to. The test can only be used when sample size is small compared to the population about which you are trying to make an inference.
Changes to the shape of a Binomial distribution at varying values of the proportion of successes, p, and number of trials, n.
The Binomial test is derived from the Binomial distribution, which can be thought of as the distribution that is followed by the number of ‘successes’ or ‘failures’ in a certain number, n, of repeated independent experiments or ‘trials’. In more statistical language, we can say that the distribution relies on the values of n and p (the probability of any trial being a success), and that these are the parameters of the Binomial distribution. It is useful to note that as the sample size (the value of n) increases, the distribution becomes more symmetrical and converges to a Normal distribution.
Binomial test assumptions
Assumptions for the Binomial test are as follows, and can be easily remembered using the ‘BINS’ acronym:
- B – the variable of interest should be a binary outcome meaning it can take only one of two values (e.g. a coin toss (heads/tails), presence of a disease (yes/no), morality (dead/alive)). This is sometimes also referred to as a dichotomous variable.
- I – observations should be independent, meaning that one observation should not have any bearing on the probability of another.
- N – the experiment should have a fixed sample size denoted n.
- S – all independent observations should have the same probability of having the outcome. This is similar to the independence assumption and can be achieved through random sampling.
Binomial test example
Suppose a population health researcher carries out a small random sample survey to estimate the prevalence (the proportion of a population affected) of herpes simplex virus (HSV), a common viral infection that causes genital and oral herpes. Members of the sample are selected at random with a total of 20 people selected (n=20), are independent from one another and have the same probability of having the outcome, and with a binary outcome of interest (presence of HSV; yes/no).
- The null hypothesis (H0) is that the proportion of survey participants (30%) with HSV is equal to 20% (0.2).
- The alternative hypothesis (H1) is that the proportion of survey participants (30%) with HSV is not equal to 20% (0.2).
We can thus conceptualize this as a series of 20 independent trials with the proportion of people with the infection, p, following the Binomial distribution. Suppose in the survey it was found that 6 (30%) of the 20 participants had HSV. The probability of a given survey participant having the disease is therefore p=0.3. Suppose also that a previous survey found the prevalence of HSV to be 20% (this could be from the same population or a comparable population) - the researchers use this as the hypothesized value on which to run the Binomial test for the current survey proportion.
The next step is to run the Binomial test and generate a p-value, which denotes the probability of getting the proportion of people with HSV as extreme or more extreme than what was observed if the true p was equal to the hypothesized value. Statistical packages such as Stata, SPSS or R Studio can be relied upon to generate the Binomial test p-value, but for illustrative purposes the formula is detailed below. If we have n independent trials with probability of having HSV being p we can calculate the probability of the value being the hypothesized number of HSV cases, r (in this case r=4 as 20% of 20 is 4), using the following formula:
By plugging the values into the Binomial formula we get 0.196, the probability of 6 or fewer HSV cases out of 20 (one tailed test). Since our hypothesis of interest is whether the observed and hypothesized values differ in any direction, we would like to generate a two tailed test, and so we multiply by 2 to get a final p-value of 0.392.
The Binomial test formula features factorials represented by an exclamation point. These are calculated by multiplying the number by itself and then by every whole number through to 1. See here for a full hand calculation of the Binomial formula, and here for a convenient online calculator.
Using a significance level of α=0.05 we fail to reject the null hypothesis because p > 0.05 and conclude that there is no evidence of a statistically significant difference between the prevalence of HSV in the current survey compared with the previous survey given the sample size.
Elliot McClenaghan is a research fellow in Epidemiology and Medical Statistics at the London School of Hygiene & Tropical Medicine