Ten Guidelines for Adopting Ontologies To Create FAIRer Scientific Data
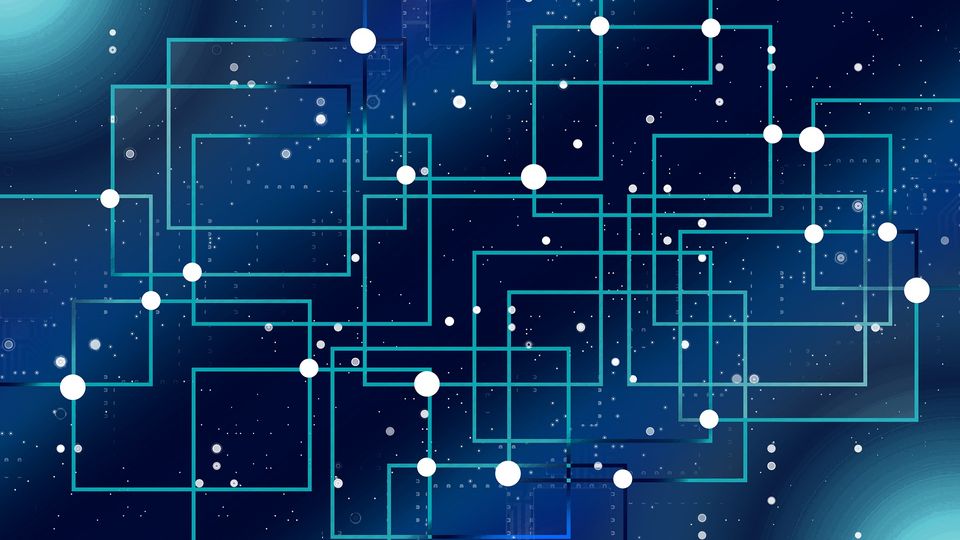
In a previous article, I looked at how ontologies can help tackle some of the life sciences’ big data challenges by FAIRifying data, making it Findable, Accessible, Interoperable and Reusable. Ontologies – human-generated, machine-readable models of a domain – can help to make data FAIR and usable from the point of creation. This reduces the time scientists spend searching for information, avoids duplicated experimental work, and makes data “machine ready” to power AI and machine learning projects.
However, deciding to implement ontologies into your data management practices can be daunting. It can also be a difficult sell to business stakeholders as the ROI is often not immediate. In this article, I outline the challenges and offer 10 guidelines for kickstarting your ontologies journey.
A business, cultural and scientific challenge
Implementing any ontology is a specialized task. Successfully doing so requires data collated from many sources to be consistently formatted, structured and harmonized. In any data-heavy field this is a challenge. But in the life sciences this challenge is particularly acute – with data sources including published literature, experimental data, and patient and clinical records, containing graphs and tables, biomedical images, social media data and voice recordings.
Life science organizations must also consider business and regulatory requirements. Companies want to ensure any ontology complies with strict governance processes and has robust version control to give a visible audit trail, whilst also needing a system agile enough to make changes easily. Building a network of ontologies that can simultaneously allow these levels of flexibility and control is difficult and time consuming.
In addition, there is growing demand for ontologies to be more “democratic” by allowing a range of users across the business to contribute to their development. This widens the pool of knowledge that feeds an ontology, so it is more accurate and reflects the needs of its users. However, this requires a shift in cultural mindset – no longer “it’s my lab and my data”; rather “it’s the company’s data and its FAIR”.
The final, and potentially most critical, challenge is proving the value of ontologies and FAIR projects to stakeholders. As with any large-scale, complex project, ROI is medium-to-longer term, and ontology projects may be at risk in the short -term. Therefore, to maximize the success of your ontology project, these are 10 things to bear in mind:
1. Uncover what’s in place already
Before planning a new project, data teams should identify what ontologies are already being used within their organization – be that public ontologies or bespoke terminologies created in-house. Building on existing work accelerates progress and provides early wins to present to stakeholders.
- Rebuild, reuse, recycle
Work on life science ontologies has been ongoing for many decades, which means there is an existing open-source framework to draw on. Public ontologies, such as MeSH from the NIH are a great starting point. Using what is already available as a foundation for your own ontologies is an easy way to make tangible progress.
- Find your FAIR champions
The companies I’ve known to have the most success are those who have “FAIR champions” who understand the challenges discussed above. FAIR champions don’t have to be experts in semantics or data science, they need to be tenacious, committed to the project and capable of enthusing stakeholders around goals and milestones.
4. Create a URI strategy
Uniform Resource Identifiers (URIs) should be established at the start of any ontologies journey. URIs provide a means of locating and retrieving resources on a network – similar to web address URLs. URIs are difficult to change once they are in place as they denote the unique ID for an entity. A common URI strategy from the outset reduces the chance of error and increases standardization across the business.
- Map sparingly
Mapping ontologies is a time-consuming and unending task, with a constantly moving target as ontologies evolve with our understanding of the life sciences. Try to limit mapping wherever possible by limiting to a small number of ontologies (ideally one!) per domain and not bringing in or creating a new ontology where one is already in use for that area.
6. Simplify your ontology selection
Minimizing the number of ontologies used reduces the burden of having to keep them in sync, or map between them. Selecting public ontologies further simplifies integration of public and private data. For example, if your domain is diseases, you might use Mondo Disease Ontology to reduce your workload.
- Start small and iterate
You can’t tackle all of your data at once. It takes too long to see returns and besides, it’s probably impossible. Start with one use case at a time – prototyping to see what works and using those learnings to iterate. Data entry projects such as assay registration are a good starting point as they already have a particular structure. It could be a simple swap from entering free text to choosing from a drop-down list of assays from your domain ontology of choice. This makes data FAIR from the outset; a standard list ensures the information is consistently recorded, interoperable and facilitating future reuse.
- Don’t let the scale of the problem put you off
An organization doesn’t need a model of the entire strategy before getting started on an ontology project. As mentioned, iterative successes are key. For example, consolidating lists of terms and uploading them centrally where people can contribute, or starting in an area that you know already has relatively good data management that can be built on to show value quickly.
- Find the business value
One of the challenges with any data management undertaking is that business value is medium to long term. To win funding and ensure the project moves forwards, find the short-term impacts and link them to business outcomes. For example, show that applying an ontology to bioassay creation has reduced time spent searching for data by X number of hours. Or show that using an ontology has made it possible to reuse valuable datasets that were previously siloed away. Tangible results must be shared early and often with business leaders.
- Empower subject matter experts
It’s essential to empower and trust your subject matter experts. That includes both your data scientists and your domain experts who can provide you with the relationships and knowledge of the field to develop the ontology properly. Give them the right tools to do the job, and a realistic time frame in which to deliver.
Driving future innovation
The use of ontologies in data management is fundamental to driving future innovation. Transformational life science leaders are spending time and resources on embedding robust data practices. They know that when scientists are able to make efficient use of data being produced, the path to new discoveries is accelerated. False starts, dead ends or being on the wrong track are more common than they need to be. This can be demotivating and frustrating.
Shortening the drug discovery lifecycle is not just valuable in terms of shareholder value and benefit to patients, it will also improve team productivity. Scientists are more engaged when they are confident that the path they are pursuing will either eventually be successful or will “fail fast”. With the right strategy and expertise, organizations can use ontologies to ensure they are at the forefront of new breakthroughs.