Recent Advances and Applications of Single-Cell Sequencing
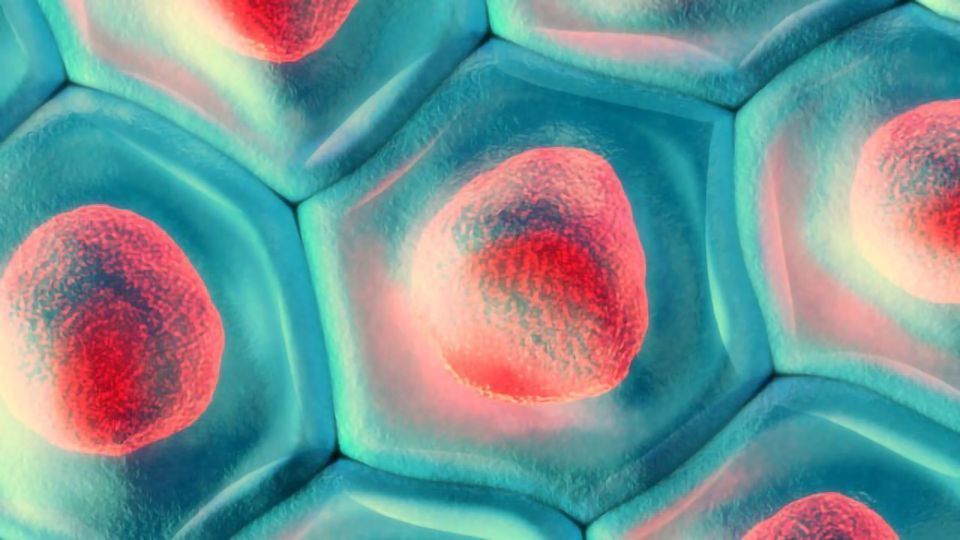
Complete the form below to unlock access to ALL audio articles.
Single-cell sequencing, or single-cell genomics technologies, have evolved rapidly in the last 10 years and have now cemented themselves as ubiquitous techniques utilized by researchers in multiple areas of biology and biomedical sciences. These techniques offer significant advantages to previously used sequencing approaches – termed bulk sequencing – which focused on analyzing the molecular characteristics of collections of cells in a tissue, rather than individual cells.
Since tissues and organs are composed of heterogenous cell types with unique molecular profiles, capturing single-cell molecular profiles allows for a deeper understanding of normal physiological processes as well as diseases. Single-cell genomics significantly adds to our understanding of how specific cell types differentiate and mature in different organ systems and how pathologies affect specific cell types. "Studies of diseases affecting the brain especially benefit from single-cell genomics technologies,” notes Lucas Schirmer, assistant professor at the University of Heidelberg and a clinician-scientist specializing on multiple sclerosis. “This is due to the tremendous cellular heterogeneity of a brain disease is very hard to resolve using other methods”.
Over recent years, single-cell sequencing has expanded beyond the boundaries of the most widely utilized techniques, such as single-cell RNA sequencing (scRNA-seq), and now permits analyzing genomic DNA, protein expression and epigenetic signatures at the single-cell level. “These diverse modalities, especially epigenetic regulation, are crucial to profile in the context of cancer since tumor cells employ various mechanisms to evade body defenses” says Dr Nicole Salazar Velmeshev, assistant professor at the San Francisco State University who studies cancer genomics. Another recent trend is simultaneous analysis of multiple molecular modalities, such as the RNA and epigenome, in the same single cell. The explosion of single-cell genomics studies would not be possible without the development of technologies for faster, more sensitive and larger-scale analysis of single cells, as well as concomitant development of bioinformatics analysis techniques. Here, we review the most commonly adopted technologies and workflows available for single-cell sequencing and discuss current research studies adopting such approaches.
Experimental workflows for single-cell RNA sequencing
scRNA-seq is the most widely used single-cell genomics technique that aims to obtain gene expression profiles of individual cells isolated from a sample of interest. Gene expression profiles are indicative of both the cell's identity and its state, and so scRNA-seq can be used to determine the type of cell that is being analyzed and how its gene expression profile (also called transcriptome) changes in a condition of interest – for example, in a specific disease state.
The first step of scRNA-seq is capturing individual cells to perform molecular barcoding of RNA molecules that originate from the same single cell. Over the last 10 years, several cell capture methods have been developed; the main approaches include capturing cells in microwells of a microfluidic device, sorting individual cells into wells of a plate using fluorescence-activated cell sorting (FACS) or, most recently, encapsulating single cells into aqueous droplets formed in a lipophilic medium. Once individual cells are captured, reverse transcription (RT) is used to generate complementary DNA (cDNA) from the RNA molecules of each individual cell, whilst simultaneously introducing a unique DNA sequence into each cDNA molecule (cell barcode). In droplet-based scRNA-seq, RT primers with cell barcodes coat a silica bead that is captured in a droplet together with an individual cell, thus ensuring that each unique cell barcode is restricted to only the RNA contained in a specific cell. In addition to the cell barcode, RT primers can also be used to include a second barcode – a unique molecular identifier (UMI) – which is unique to each DNA molecule of the primer. UMIs allow the quantification of the number of original RNA molecules, rather than cDNA, which is preferential because the number of cDNA copies is affected by technical factors, such as the PCR bias. cDNA is then prepared for next-generation sequencing (NGS) by fragmenting it, ligating sequencing adapters and amplifying it. By sequencing the cell barcode, the UMI and the portion of cDNA corresponding to the original RNA molecule, you can determine which individual cell each RNA molecule belongs to and quantify its abundance.
Workflows for other single-cell genomics applications
Workflows for other single-cell sequencing methods, such as single-cell epigenetics profiling, usually involve either the sorting of individual cells into wells of a plate or a method termed combinatorial indexing. Combinatorial indexing uses a set of two barcodes, rather than a single cell barcode, to label RNA or DNA originating from the same single cell and provides single-cell resolution without the need to sort individual cells. Once the biomolecules are barcoded, they are processed according to the desired readout, such as transposase digestion for open chromatin analysis with single-cell ATAC sequencing (scATAC-seq) or bisulfite treatment for single-cell DNA methylation analysis.
Novel methods have recently been developed to profile multiple molecular modalities, such as open chromatin and RNA expression, in a single cell. “Combinatorial assays are especially attractive to us as cancer biologists as they open avenues in studying both the type and state of tumor cells, as well as their epigenetic status. Some of the most effective cancer treatments are directed at modulating epigenetic state of tumor cells, so understanding cancer epigenetics is extremely important for future treatments,” says Velmeshev.
Incorporating spatial mapping and confirmation of gene signatures into single-cell RNA sequencing workflows

Complex, highly heterogeneous tissues, such as the brain, are comprised of multiple cell types and states. However, interrogation of these complex tissue types requires a highly sensitive, specific, and multiplexed spatial approach with single cell resolution. Download this app note to discover a technology that rises to these challenges, enabling researchers to detect up to 12 RNA targets simultaneously. As well as spatially map scRNA-seq gene profiles at the single cell level in the tissue context and localize newly identified cell subtypes and cell markers.
Download App NoteSponsored Content
Bioinformatics analysis of single-cell sequencing data
Once scRNA-seq data is generated, several options are available for downstream bioinformatics analysis. First, the raw sequencing reads need to be aligned to the reference genome, cell barcodes need to be detected and reads (or UMIs) originating from the RNA of the same cell need to be assigned to genes and quantified. This section of scRNA-seq analysis is computational resource-intensive and is usually performed on a high-performance compute cluster. Several free bioinformatics pipelines, such as dropSeqPipe, exist for analysis of raw data generated using Drop-seq and plate-based scRNA-seq methods.
The end result of the analysis of raw scRNA-seq data is a matrix of read or UMI counts per gene for each cell captured and analyzed. This matrix is the input to downstream analysis tools that aim to identify specific cell types contained in the original sample, their gene expression markers, as well as transcriptomic changes in cell types in experimental conditions. The most ubiquitously used tools for downstream analysis of single-cell genomics data are software packages Seurat1 and Monocle2. Seurat can be used to perform unbiased clustering of single-cell genomics data, which groups cells based on how similar their single-cell molecular profiles are. Seurat can also be used to identify markers of resulting clusters (which often correspond to cell types), in addition to integrating datasets from different sources and modalities (e.g. scRNA-seq and scATAC-seq). Monocle is utilized to perform so-called pseudotime analysis, which arranges single cell profiles according to dynamic changes in gene expression or other modality, such as ATAC-seq signals. Pseudotime analysis is instrumental in discovering changes in specific cell types that happen over a period of time or as a result of a continuous process, such as brain development or neural degeneration. Importantly, both software packages can be applied to different modalities of single-cell sequencing data and are not restricted to scRNA-seq.
Large-scale genomics studies leveraging scRNA-seq
scRNA-seq has been applied to a number of biological questions; however, this technique has been especially successful at tackling questions in the area of neuroscience. This is due to the fact that the mammalian and especially the human brain is arguably the most complex organ consisted of tens to hundreds of diverse cell types. Unlike cells in other organs such as the immune system, brain cells are hard to isolate using conventional techniques like FACS.
scRNA-seq has been used to create a single-cell molecular atlas of the developing human brain, which created a blueprint for human brain development that is crucial for understanding of diseases of nervous system development. In a series of high-profile studies, a modification of scRNA-seq termed single-nucleus RNA sequencing (snRNA-seq) has been applied to post-mortem brain tissue samples to study molecular changes in specific cell types in neurodevelopmental and neurodegenerative diseases, such as autism spectrum disorder3, multiple sclerosis4 (MS) and Alzheimer’s disease5. Schirmer, the first author on the MS snRNA-seq study, notes: “Single-nucleus RNA-seq has really been an eye-opener for us in regard to the MS pathology. Just five years ago, we were only able to study expression of a handful of genes in a limited number of cell types. Now, using the same tissue, we can know how every gene changes in each cell type over the course of MS lesion progression.”
Latest studies that utilized single-cell epigenetics profiling
Single-cell epigenetic profiling is quickly becoming instrumental in uncovering how specific cell types in tissues and origins develop and are affected by disease. As such, scATAC-seq has recently been used to investigate how epigenetic signatures of cell types in the developing human brain6 change as they differentiate and mature. Additionally, scATAC-seq has been used to gain insight into the role of epigenetic regulation in the progression of various cancers, such as triple-negative breast cancer7. According to Velmeshev, “These novel approaches, including single-cell ATAC sequencing and DNA methylation, will be allow us to look how specific clones of tumor cells change their epigenetic profiles and how these changes can be corrected by epigenetic drugs”.
Over recent years, single-cell sequencing has become a method of choice in many areas of biomedical research due to its ability to provide information about the molecular state of all the cell types and thousands of individual cells in a sample. These techniques are developing rapidly to improve their sensitivity and scalability and to reduce price, time and resources required to carry out single-cell sequencing studies.
References:
2. Cao J, Spielmann M, Qiu, X et al. The single-cell transcriptional landscape of mammalian organogenesis. Nature. 2019;566(7745):496-502. doi:10.1038/s41586-019-0969-x
** This article is based on research findings that
are yet to be peer-reviewed. Results are therefore regarded as preliminary and
should be interpreted as such. Find out about the role of the peer review
process in research here.
For further information, please contact the cited source.