Oncology Researchers: Are You Getting the Most From Your Digital PCR Data?
Learn what ideal dPCR data looks like and how to deal with bad data.
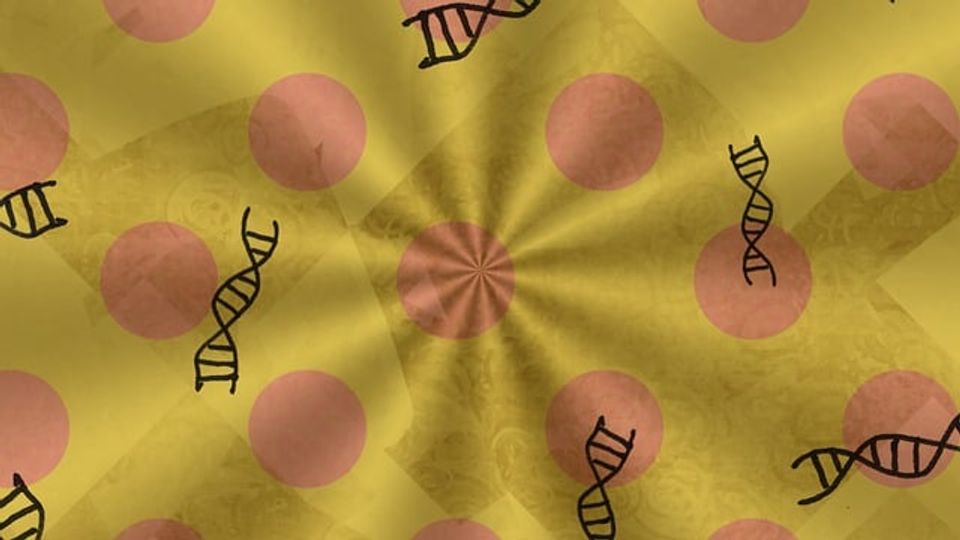
Complete the form below to unlock access to ALL audio articles.
Cancer can seemingly change in the blink of an eye, making detection and monitoring incredibly time-sensitive. For fast, sensitive and accurate testing for tumor DNA, cancer researchers are increasingly relying on digital PCR (dPCR). However, when making these measurements, there's little room for error. Here we'll discuss what good dPCR data looks like, how substandard equipment can detract from data quality and what oncology researchers can do to get high-quality dPCR data that will provide accurate and efficient insight into tumor load.
Only the best: DNA testing must be top-notch
For many cancers, the sooner treatment begins, the larger the treatment window is and the more likely a patient is to survive. However, based on a tumor’s genetic makeup, some treatments may be more effective than others. Next-generation sequencing can accelerate the selection of appropriate treatment by identifying molecular biomarkers in the tumor driving the disease or rendering it drug resistant.
Subsequently, many researchers are exploring the use of a noninvasive technique called a liquid biopsy to track these biomarkers. This technique assesses circulating tumor DNA (ctDNA) to measure biomarkers in the blood and other bodily fluids. Liquid biopsy data can give insight into tumor load, indicate prognosis and guide treatment decisions about when to administer a chosen therapy and for how long. These insights have significant potential to enable more effective, personalized cancer treatment in the future.
ctDNA occurs at minuscule levels in liquid biopsy samples, so it is critical to use highly sensitive technology, such as dPCR, to ensure accurate results. However, when comparing the numerous dPCR systems available, different instrument designs produce data of varying precision, accuracy and sensitivity. Inconsistent data undermines the overwhelming successes seen in biomarker research as a whole. Ultimately, for liquid biopsy to shift into standard clinical use, oncology researchers need tools and reagents that can be relied upon to produce the highest quality biomarker data possible.
Different data from different instruments
All dPCR approaches are designed to achieve extremely accurate and precise nucleic acid testing, providing absolute quantification of target species. A dPCR assay involves partitioning a sample into thousands of separate units, each containing one or a few DNA strands. This can be done on a microarray, on a microfluidic chip, in quantitative PCR-like microfluidic plates or, in the case of Droplet Digital PCR (ddPCR), in an oil-water emulsion. Next, a PCR reaction occurs in each partition and fluorescent probes amplify the target nucleic acid. At the end of the reaction, partitions are evaluated for positive or negative fluorescence. Researchers use Poisson statistics to determine the concentration of target nucleic acid in the original sample.
In contrast to quantitative PCR (qPCR), dPCR assays do not require researchers to run their samples alongside a standard curve to interpret results, reducing the possibility of human error. It also makes the high sensitivity and high limit of detection of dPCR assays possible, rendering them capable of assessing samples containing less than one percent of a target species – which is often the case for liquid biopsy samples.
As with any technology, the type of instrument, quality of reagents and user ability all affect the reliability of a liquid biopsy assessed with dPCR. Because certain elements of dPCR instruments and assays vary, not all instruments will produce data of the same quality. While it's preferable to choose a system that produces results at low cost, with a simple workflow and low sample-to-results time, researchers must also prioritize the quality of ctDNA testing to select an instrument that will add real insight to their research.
The bottom line: researchers can only act on biomarker data if they can trust the results of their dPCR assays. So, what does good data look like?
How to distinguish between good and bad data
dPCR data should be binary, meaning that positive and negative points on a graph are clearly separated by a threshold (Figure 1). Bad dPCR data sets have a poor separation between positive and negative partitions and may display a great deal of noise, making a threshold challenging to place. Bad data may result from several issues, such as a faulty assay or instrument or an inhibitor affecting the assay. When this happens, the researcher must repeat the assay, delaying the critically time-sensitive information about how a tumor responds to treatment and costing additional money.
Good Data | Bad Data |
|
|
|
|
Bad data appears in various forms that may provide insight into the source of the trouble (Figure 2). Attempting to interpret any of these kinds of data comes with a high risk of inaccurate quantification.
● Random positive partitions appear dispersed like rain on the graph. This issue occurs when the target has amplified DNA at a variable rate across partitions. It may indicate a low-specificity assay, poor sample partitioning or instrument noise. Because positive and negative partitions blend together, it isn't easy to draw a threshold, which reduces the limit of detection.
● Hardware instability may lead to inconsistent amplitudes within positive and negative partitions, making it unclear where to draw the threshold. An experienced user must attempt to draw the threshold and interpret results manually to make sense of the results. However, this introduces the possibility of human error, thereby reducing sensitivity.
● Noise and optical instability cause data with negative data points to cluster above the threshold. This kind of data usually results from bubbles, solid contaminants or optical issues with the instrument. Again, a well-trained user can manually make sense of these results, but the process takes up a researcher's valuable time and introduces human error, lowering assay sensitivity.
● Cross-contamination occurs when the target DNA moves from one partition to a neighboring one. This issue may arise from user error, environmental factors or sample dispersion caused by the instrument itself. Cross-contamination causes negative partitions to appear truly positive, making it impossible to trust results. Researchers may be able to determine if cross-contamination has affected their assays by looking at the no-template controls that should not produce positive data points. dPCR assays that are run on microarrays and plate chips are particularly prone to this issue, while ddPCR assays performed in oil-water emulsions are not.
Why is good data important for ctDNA testing?
Highly promising research areas aim to use ctDNA testing throughout cancer treatment: during neo-adjuvant therapy to rapidly assess tumor status and drug efficacy, after curative procedures to measure the residual tumor burden and during long-term monitoring to catch tumor recurrence as early as possible. For this research to proceed unhindered and become a standard in clinical care, it's crucial to use technology that will generate the highest quality data to determine the utility of each of these approaches.
By turning to high-quality instruments, reagents and methods to advance their work, oncology researchers can proceed confidently, keeping pace with the time-sensitive nature of cancer treatment. This way, they can ensure that their work will continually improve the standard of care for future cancer patients.