New Frontiers in Pharmacokinetics
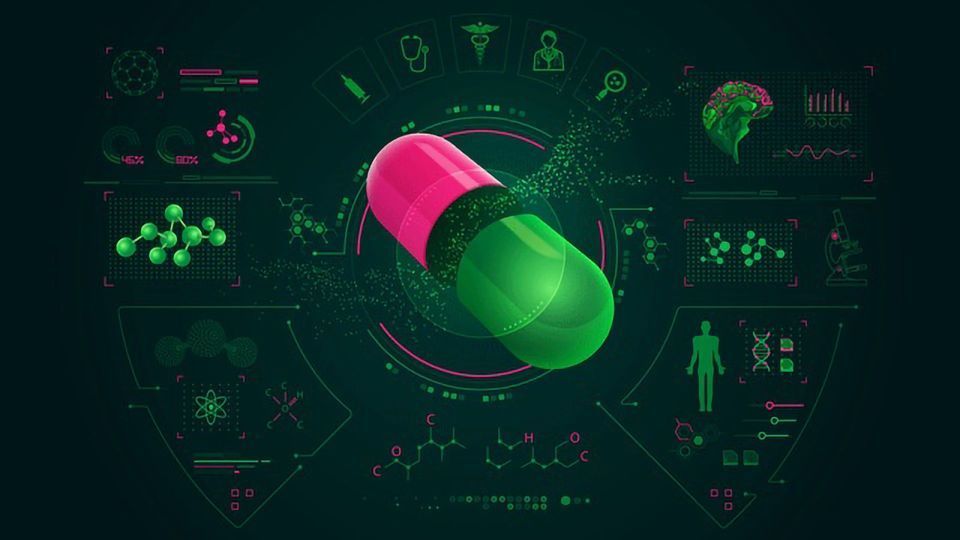
Complete the form below to unlock access to ALL audio articles.
Today, we have tools to predict how a certain type of drug will be absorbed, distributed, metabolized and excreted by the body (its ADME properties) thanks to decades of research on pharmacokinetics. Yet, most of our human pharmacokinetics data comes from clinical trials conducted in homogenous cohorts of people, chosen based on strict eligibility criteria. Clinicians have to select the most appropriate treatment and dose for individual patients based on “averages”. In this article, we look at two factors that may impact these averages – our gender, and the microbes we share our body with.
Understanding drug–microbiome interactions
The liver is the main site of drug metabolism, and we already know a lot about how the liver will transform drugs and have a good understanding of the enzymes involved. Yet when it comes to individual drug response, there is another player involved – our microbiome – and our understanding of its impact on drug pharmacokinetics is much more limited.
“This is partly because the microbiomes of preclinical animal models are quite different from the microbiomes of humans,” explained Andrew Goodman, C.N.H. long professor of microbial pathogenesis and director of the Microbial Sciences Institute at Yale University, “and although we know that the microbiome is highly variable between individual people, typical early human studies of drug metabolism don't include the wide range of individuals that would capture more of this microbiome variation.”
The human microbiome encodes at least one hundred-fold more genes than the human genome, and Professor Goodman’s team is on a quest to find out how these microbes and their genes impact drug metabolism, and vice versa. In one recent study,1 they looked at 76 different microbes that are prominent in the gut and their effect on 271 US Food and Drug Administration (FDA)-approved oral drugs, and for two thirds of the drugs, at least one gut microbe could significantly metabolize the drug.
“What we have learned so far is that the capacity of gut microbes to metabolize drugs is much broader than we had anticipated,” said Goodman. “Then there's an added level of complexity, for example in cases where two microbes metabolize the same drug, but in a different way. We're just scratching the surface of understanding of how these microbes are achieving this and, just as importantly, understanding in what circumstances this will matter in people.”
With so many complicated drug–microbe interactions, Goodman is prioritizing answering basic questions to lay the building blocks of knowledge in this field in the same way that was done for liver metabolism over several decades. “At this point, we can't look at a drug and say this is how these microbes will metabolize the drug. Our approach is to try to identify the genes and the enzymes that these microbes are using, because we think these are going to be the informative biomarkers that predicts how a drug will be metabolized, rather than the microbe itself.”
To do so, Goodman’s lab is developing models in germ-free animals where they can manipulate whether or how the animals’ microbes contribute to drug metabolism.2 “We have animals that are genetically identical, except for one enzyme in their gut microbes, and then we follow a drug’s journey not only through the gut but through the whole body, using pharmacokinetic approaches to understand what contribution microbial activity makes to systemic drug exposure.”
The goal is to answer two questions: what is the chemical transformation behind the microbial metabolism of certain drugs and what are the effects of these metabolites on the body? “For example, do these metabolites affect liver metabolism of the parent drug by competing for the same substrate? Or do they cause local effects in the gut?” explained Goodman. “Then, it's going to be very important for us to connect what we're studying in these simplified systems to studies in humans.” Goodman plans to identify cohorts of patients taking certain drugs and study their microbiomes beforehand, to see if their microbiome composition helps us better understand drug individual pharmacokinetics.
There are several ways this information will be useful in future: “In the drug development phase, it might be important to use the right preclinical model that captures microbial activities that may be happening in humans,” said Goodman. “Then in early clinical trials you would ensure that participants represent relevant microbiome activities, so you can see those effects before getting to a very large clinical trial where you're only going to start collecting those individuals by chance.”
For drugs that are that are already in use, some of the same approaches that are used in pharmacogenomics could also apply here. “If we understood how an individual's microbiome will impact their drug response, potentially we could make a better choice of which drug or which dose an individual should get,” said Goodman. There is a key difference though. “It's reasonable to think that in coming years we'll be able to give treatments that transiently modify a person's microbiome to improve their response to a drug in a way we would be unlikely to try to change somebody's genome.”
Binding Kinetics: High-Throughput Assay for Kinase Inhibitors

Efficacy and safety of drug candidates is dependent on their binding kinetics – how fast they associate (kon) and dissociate (koff) from their targets, and for how long they can occupy them. Ideally, these kinetic parameters should be determined during the preclinical stages of development, creating the need for high-throughput methods to profile hundreds of compounds in an efficient way. Download this app note to discover a high-throughput assay that aids the selection of new drug candidates for development according to their binding properties.
View App NoteSponsored Content
Identifying gender-specific adverse events
Nicholas Tatonetti is associate professor of Biomedical Informatics at Columbia University, where they focus on making drugs safer through data analysis. They recently developed a tool called AwareDX that can identify risk of adverse events that predominately affect women.3
“It’s a quirk of science history that most studies on drug effects have been done in men,” said Tatonetti. “Often when researchers were originally doing model organism studies, they focused on using male mice or rats because they thought there might be cyclical hormonal changes in female animals that would confound the study. This was a naive assumption because we know that male mice, rats and humans all have cyclical, hormonal fluctuations as well. But I think this mindset translated up to clinical trials, and until fairly recently clinical trials were only done in healthy young white males, producing all types of biases leading to unexpected and severe events.”
Payal Chandak, a senior computer science major in Tatonetti’s lab, was motivated to analyze these biases after shadowing a doctor during her studies. “I found an example of an insomnia drug called Ambien, which was first approved in 1995, but then 21 years later, the dose had to be halved in women when it was found they were experiencing a stronger reaction to it – they were really drowsy in the morning and were getting into a lot more car accidents than men.” Although there's a few clinically established examples like this, there was, until now, no systematic understanding of gender-specific adverse effects, even though the information would be very valuable to doctors prescribing drugs.
To address this, Chandak and Tatonetti built an algorithm that takes details of adverse events from the FDA Adverse Event Reporting System (FAEERS) and removes the confounding biases that make it challenging to identify authentic gender-specific adverse events. “If you were to just run a simple statistical analysis on that raw data, asking: “Do women have this adverse event when they're on this drug more often than men?” it would be influenced by many biases because so many diseases and drugs are sex specific, like prostate cancer or contraceptives,” explained Chandak. “What our model does is use machine learning to correct for many of those biases and build balanced cohorts of reports from this database. Then on those balanced cohorts, we apply statistical analysis to answer that question.”
The result is an openly available resource of 20,817 adverse drug events that the algorithm predicts pose sex-specific risks to both women and men, covering 792 unique drugs. To validate their algorithm, they used well-established adverse events linked to pharmacogenetics and tried to recall the same results. “The main discovery we made was that these gender-specific adverse events are very nuanced,” said Chandak. “For example, AwareDX correctly showed that a drug transporter gene called ABCB1 has different downstream effects in men and women.” Men with the gene are at risk of myalgia from a drug called simvastatin, whereas women with the gene are at risk of long QT interval with a drug called risperidone – completely different risks linked to the availability of one gene in liver cells. “It shows that it’s very important to understand the genetic basis and nuances that go along with these sex differences, rather than just simply saying that this drug is dangerous for women or for men.”
At this stage, the tool is intended for research purposes rather than clinical decision-making and the goal is to trigger more scientific discussion around gender differences in adverse events. “The associations that we find are still just associations and they need to be further examined and proved out,” said Tatonetti. “I think it's really interesting for pointing to the differences in mechanisms between men and women and how they metabolize drugs and how they respond to drugs.” In addition to providing the basis for mechanistic studies, the data could be used in retrospective analysis of electronic health records. “Once this information is studied further and corroborated, it’s actually going to impact drug prescriptions and people’s health.”
Enhanced Productivity and Labor Efficiency in Lot Release and In-Process Testing of Biologics in GxP Laboratories

Bio-Layer Interferometry (BLI) is a technique used for analysis of real-time biomolecular interactions and can be used in assays such as the quantification of antibodies and recombinant proteins. This is a significantly useful technique, as it can be used as an alternative to ELISA and HLPC methods and provides a reduced workload with higher throughput results. Download this whitepaper to understand the benefits of BLI technology, including how BLI can provide highly precise results and the cost-effectiveness of using BLI from decreased workload.
Download WhitepaperSponsored Content
Towards predictive individualized pharmacokinetics?
Despite all we have in common, no two humans are biologically the same, and these studies highlight just how variable drug properties can be from person to person. They also show that through a combination or experimental and computational approaches, we can develop promising tools to identify common factors – genes, microbes, or clinical features – that will help predict a person’s pharmacokinetics in the real world.
References
1. Zimmermann, M., Zimmermann-Kogadeeva, M., Wegmann, R. et al. Mapping human microbiome drug metabolism by gut bacteria and their genes. Nature. 2019;570:462–467 doi:10.1038/s41586-019-1291-3
2. Zimmermann, M., Zimmermann-Kogadeeva, M., Wegmann, R., and Goodman, A.L. Separating host and microbiome contributions to drug metabolism and toxicity. Science. 2019;363(6427):eaat9931 doi:10.1126/science.aat9931.
3. Chandak P., Tatonetti, NP. Using machine learning to identify adverse drug effects posing increased risk to women. Patterns. 2020;1(7):100108 doi:10.1016/j.patter.2020.100108