Interview: A Deeper Cut Into Behavior With Mackenzie Mathis
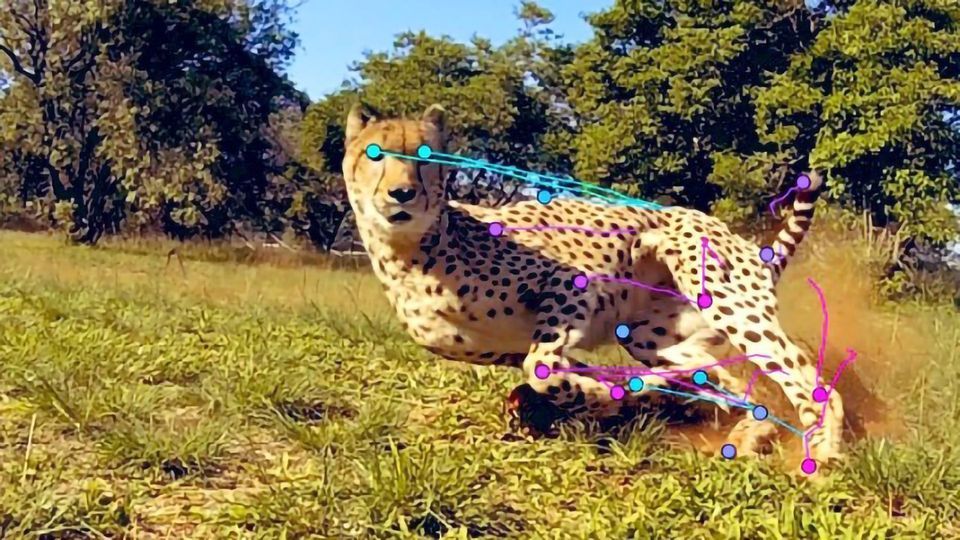
Complete the form below to unlock access to ALL audio articles.
To the untrained eye, DeepLabCut appears to do something pretty basic. It adds dots and lines to video footage of moving animals. But this technology is part of a wave of automation that is revolutionizing behavioral analysis. Don’t just take my word for it; look to the recent announcement by the Chan Zuckerberg Institute that DeepLabCut was one of 32 proposals selected for Essential Open Source Software for Science funding, which will see $5 million go towards vital software projects that have been designed with access and community in mind.
In this interview, conducted at the Neuroscience 2019 conference, we talk with DeepLabCut’s co-creator, Dr Mackenzie Mathis, a principal investigator at Harvard’s Rowland Institute, about open science, DeepLabCut’s potential for prosthetics, and how to safeguard against automated software being used for the wrong reasons.
Ruairi Mackenzie (RM): What can we learn about the brain by studying how the body moves?
Mackenzie Mathis (MM): From my perspective, which is very centrally focused on the core tenets of the computations of the brain, behavior is the final output, so it’s the most important thing that the brain does. In particular, I’m in the motor control field and so, as a nod to Daniel Wolpert, the only reason we have a brain is to move, right? The sea squirt ingests its own brain when it finds it rock that it wants to stay on. But not being so tongue in cheek, the reductionist approach to behavior is really powerful but I think there’s a modern resurgence of understanding that there’s a lot of complexity to behavior. Being able to open a toolbox to quantify complicated behaviors is really fruitful, if you’re trying to understand how the brain might be involved.
RM: How do we infer back from the movements to what the animal’s intention is?
MM: That depends what your definition of intention is. I guess if we keep it into terms of neurons there’s a lot of studies that take what you can measure from the outside, like the movements of the animal. You can correlate that to neural activity and perturb neural activity to see how behavior changes with high fidelity. Even historically that was quite complicated without computer vision. It was manually labor-intensive to do this type of work. It was done a little bit less because it was so expensive to do.
With intention, of course, you get more into this notion of “What are the animal’s internal states that drive behavioral actions?” and so in that sense the tools that we develop are more or less trying to take the human out of the equation in terms of the manual labor aspects. Then there’s this whole other world of what do you do with that data.
RM: Where does DeepLabCut come in?
MM: DeepLabCut is essentially a tool that replaces the human in terms of the labor of labelling data. Pose is by definition a geometric configuration of body parts, so you can label whatever you want to label, as long as you can see it you can track it. If you need to, you can analyze the behaviors after tracking the animal; this is typically what people want to do. It’s nice to make into cool videos but at the end of the day we care about understanding behavior and its relationship to the brain. We see DeepLabCut as a module that allows this to be automated, making for more robust and reproducible science on sharable networks. These are really important factors. There’s a lot of other modules that the outputs of DeepLabCut can then be plugged into to study behavior and kinematics and soforth.
RM: What kind of time savings are we talking about in going from human analysis to automated analysis?
MM: If I wanted to manually annotate high speed videos of a mouse reaching out and grabbing a joystick, which is one of the behaviors we study in my lab, we take videos at 250 frames per second. If you want to annotate 20 key points, you can just already start to think about how much time that would take you to click on each frame to do that. Very often people would spend six months to a year in their PhD or their undergraduate work or their Master’s thesis just annotating this data before they can actually do science with it.
Now, you can essentially go in on an afternoon, label a few hundred frames in a matter of hours, train a deep neural network in four to six hours and then you have a detector that you just run new videos in. To turn six months of work into an afternoon or day of work is some pretty big timesaving.
A case study of DeepLabCut: the software was trained on 95 images taken from this video of a brown horse... Credit: Mathis Lab/ Data and human-annotation by Byron Rogers of Performance Genetics
It was then able to automatically track footage of this new horse... Credit: Mathis Lab/ Data and human-annotation by Byron Rogers of Performance Genetics
And subsequently, with just 11 extra frames of training, track this footage of American Triple Crown-winning horse Justify. Credit: Mathis Lab
RM: Have you had good feedback from your users?
MM: Yes, it’s generally been really a wonderful experience. This was a tool that was borne out of necessity for our own research; we never envisioned it being such a large project but, in that sense, I’m very happy by the community’s uptake. I think the users are the best testament it works. It’s been really nice and reassuring to see that it is working, and people are happy with it. It’s a growing tool base and we constantly put new updates and features into it as people give us feedback and we also appreciate that not everyone is comfortable with using deep learning languages and processes so we have tried to share a ton of freely-available code to make this very accessible and easy.
RM: Was it always a straightforward decision to make it open access?
MM: Yes, that was definitely a very easy decision for us. As co-developers, my husband Alexander Mathis and I feel very strongly about open science and open data, preprints and publishing that is accessible to the people that fund the research, namely the taxpayers. It was super important to us to not have it closed source and it’s been very open. I think it also pays off a lot because people get excited about open source projects and they want to contribute and so there’s a lot of contributors building modules. If it’s closed source code it’s always in the hands of the developer and sure, you make money (which maybe is great to hire more programmers), but at the same time there’s a lot of talented researchers in the world that want to contribute. They also bring their own creativity and needs to the project, which is exciting. Typically, it’s just a matter of these open source projects getting enough attention, if you will, to launch. Obviously, there’s tons of amazing open source projects that are out there. That’s one thing we think about a lot in the larger scheme; how do we build up platforms for open source tool developers, much like the team at Open Ephys and Miniscope have beautifully done. This is their first booth at [Neuroscience 2019], and it’s been amazing just to see how many people will walk by; there’s a clog of people at their booth. It’s really exciting to see people getting behind these open source projects.
RM: Thinking a bit more about the application of DeepLabCut, I understand your lab is moving to Lausanne next year…
MM: Yes, EPFL here we come!
RM:You’ll be moving to the Center for Neuroprosthetics at EPFL. Do you see DeepLabCut having an application there?
MM: Yeah, absolutely. I think it’s going to really rewarding to be closer to labs that are actually doing translational work and certainly it’s a direction we’re interested in working in and collaborating with as well. We generally focus on a lot of basic science, circuits and systems-level questions, but I think that’s a really nice merger to get people together that are tool builders, engineers, neuroscientists, theoreticians, mathematicians and things like that to really push towards the next level. The Centre for Neuroprosthetics has been quite a powerful platform in going from rodents to humans. It’s a pretty cool endeavor.
RM: Can you elaborate on how DeepLabCut might be used for prosthetics research?
MM: I don’t know all the aims of their research programs but for example Grégoire Courtine’s team has used detailed kinematic analysis for a very long time to look at rehabilitation and movements of animals. I think one part of their process that DeepLabCut can compliment is to eliminate some of the manual labor, i.e. placing markers on animals, making it more natural to look at these animals as best you can without perturbing them and their behaviors. We also work in the space of doing online feedback between behavior and the brain and that’s an interesting space to work into as well, namely to try and build new tools and algorithms that assist them in their and our studies.
RM: With DeepLabCut’s technology, whilst it has many positive applications, I can also see that nefarious organizations, companies, governments would love to be able to analyze what people are doing to understand what they’re thinking. Are there any steps we can take to safeguard against this?
We’re still a long way off I think from being too nefarious and I think people really care deeply about these questions. At least for animals we can debate whether or not there’s ethics in tracking animals in the wild or doing things like that, of course they’re not consenting beings to some degree. Of course, as we both probably recognize the potential damage of tracking a zebra in safari is less. It’s more about conservation, not about ruining the zebra’s life.
RM: Computational neuroscience is male-dominated. The attendance at [major neural machine learning conference] NeurIPS 2016 was just 15% female. You are a young, female PI, which must be quite rare in this field.
MM: It is an interesting thing. Historically these have all been very male-dominated fields but there’s NO genetic basis that that should be the reason of course. There’s a lot of great work geared towards getting women into STEM, and getting others more involved and thinking about leaky pipelines. Also, making tools more accessible and not scary for anyone is really, really important. I’m also involved with an organization, the Science Ambassador Scholarship that particularly tries to promote young women in STEM fields, so I definitely have a passion for getting women to code and to feeling comfortable with it; you can be as good a programmer and/or scientist as anyone else, it doesn’t matter your background or your gender.
Mackenzie Mathis (pictured) was speaking with Ruairi J Mackenzie, Science Writer for Technology Networks