Collective Intelligence Can Help Reduce Medical Misdiagnoses
Knowledge engineering methods could reduce the number of misdiagnoses by combining the diagnoses of multiple clinicians.
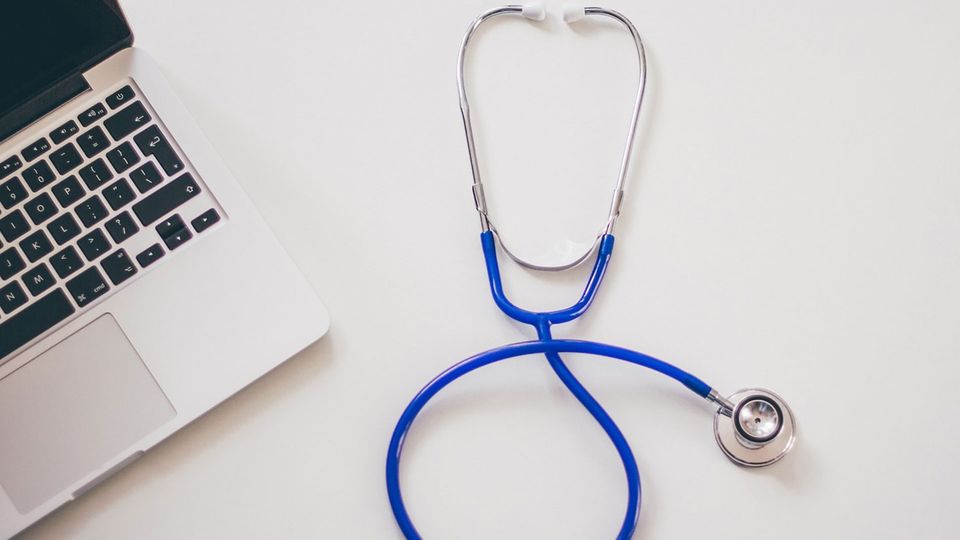
Complete the form below to unlock access to ALL audio articles.
An estimated 250,000 people die from preventable medical errors in the U.S. each year. Many of these errors originate during the diagnostic process. A powerful way to increase diagnostic accuracy is to combine the diagnoses of multiple diagnosticians into a collective solution. However, there has been a dearth of methods for aggregating independent diagnoses in general medical diagnostics. Researchers from the Max Planck Institute for Human Development, the Institute for Cognitive Sciences and Technologies (ISTC), and the Norwegian University of Science and Technology have therefore introduced a fully automated solution using knowledge engineering methods.
The researchers tested their solution on 1,333 medical cases provided by The Human Diagnosis Project (Human Dx), each of which was independently diagnosed by 10 diagnosticians. The collective solution substantially increased diagnostic accuracy: Single diagnosticians achieved 46% accuracy, whereas pooling the decisions of 10 diagnosticians increased accuracy to 76%. Improvements occurred across medical specialties, chief complaints, and diagnosticians’ tenure levels. “Our results show the life-saving potential of tapping into the collective intelligence,” says first author Ralf Kurvers. He is a senior research scientist at the Center for Adaptive Rationality of the Max Planck Institute for Human Development and his research focuses on social and collective decision making in humans and animals.
Collective intelligence has been proven to boost decision accuracy across many domains, such as geopolitical forecasting, investment, and diagnostics in radiology and dermatology (e.g., Kurvers et al., PNAS, 2016). However, collective intelligence has been mostly applied to relatively simple decision tasks. Applications in more open-ended tasks, such as emergency management or general medical diagnostics, are largely lacking due to the challenge of integrating unstandardized inputs from different people. To overcome this hurdle, the researchers used semantic knowledge graphs, natural language processing, and the SNOMED CT medical ontology, a comprehensive multilingual clinical terminology, for standardization.
Want more breaking news?
Subscribe to Technology Networks’ daily newsletter, delivering breaking science news straight to your inbox every day.
Subscribe for FREEThe researchers are currently collaborating – along with other partners – within the HACID project to bring their application one step closer to the market. The EU-funded project will explore a new approach that brings together human experts and AI-supported knowledge representation and reasoning in order to create new tools for decision making in various domains. The application of the HACID technology to medical diagnostics showcases one of the many opportunities to benefit from a digitally based health system and accessible data.
Reference: Kurvers RHJM, Nuzzolese AG, Russo A, Barabucci G, Herzog SM, Trianni V. Automating hybrid collective intelligence in open-ended medical diagnostics. PNAS. 2023;120(34):e2221473120. doi: 10.1073/pnas.2221473120
This article has been republished from the following materials. Note: material may have been edited for length and content. For further information, please contact the cited source.