An Overview of Next-Generation Sequencing
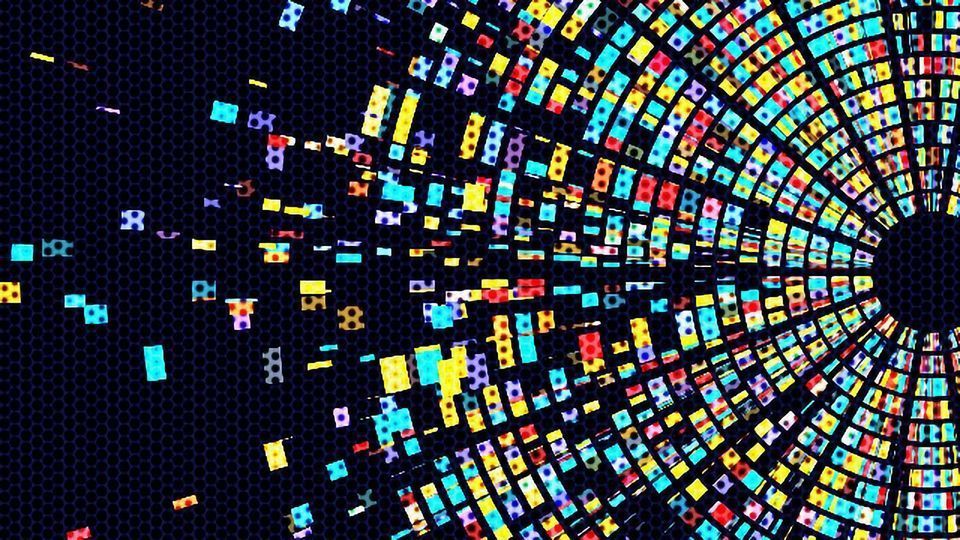
Complete the form below to unlock access to ALL audio articles.
Over the last 56 years, researchers have been developing methods and technologies to assist in the determination of nucleic acid sequences in biological samples. Our ability to sequence DNA and RNA accurately has had a great impact in numerous research fields. This article discusses what next-generation sequencing (NGS) is, advances in the technology and its applications.
- Main steps of 2G sequencing methods and next-generation sequencing library prep
What's the difference between whole-exome vs whole-genome sequencing?
Next-generation sequencing data analysis
Next-generation sequencing bottlenecks
Next-generation sequencing applications
Next-generation sequencing key terms and abbreviations
What is next-generation sequencing?
The structure of DNA was determined in 1953 by Watson and Crick based on the fundamental DNA crystallography and X-ray diffraction work of Rosalind Franklin.1,2 However, the first molecule to be sequenced was actually RNA – tRNA – in 1965 by Robert Holley and RNA of bacteriophage MS2 later on.3,4 Various research groups then began adapting these methods to sequence DNA with a breakthrough coming in 1977 by Fredrick Sanger and colleagues, developing the chain-termination method.5 By 1986, the first automated DNA sequencing method had been developed.6,7 This was the beginning of a golden era for the development and refinement of sequencing platforms, including the pivotal capillary DNA sequencer.
The chain-termination method, also known as Sanger sequencing, uses a DNA sequence of interest as a template for a PCR that adds modified nucleotides, called dideoxyribonucleotides (ddNTPs), to the DNA strand during the extension step.8 When the DNA polymerase incorporates a ddNTP, the extension ceases leading to the generation of numerous copies of the DNA sequence of all lengths spanning the amplified fragment. These chain-terminated oligonucleotides are then size separated using gel electrophoresis in early methods, or capillary tubes in later automated capillary sequencers and the DNA sequence is determined. With these immense technological advances, the human genome project was completed in 2003.9 In 2005, the first commercially available NGS platform, or second generation (2G) as it has become, was introduced, able to amplify millions of copies of a particular DNA fragment in a massively paralleled way in contrast to Sanger sequencing.10
The key principles behind Sanger sequencing and 2G NGS share some similarities.11,12 In 2G NGS, the genetic material (DNA or RNA) is fragmented, to which oligonucleotides of known sequences are attached, through a step known as adapter ligation, enabling the fragments to interact with the chosen sequencing system. The bases of each fragment are then identified by their emitted signals. The main difference between Sanger sequencing and 2G NGS stems from sequencing volume, with NGS allowing the processing of millions of reactions in parallel, resulting in high-throughput, higher sensitivity, speed and reduced cost. A plethora of genome sequencing projects that took many years with Sanger sequencing methods could now be completed within hours using NGS.
There are two main approaches in NGS technology, short-read and long-read sequencing, each with its own advantages and limitations (Table 1).13 The main scope for investing in the development of NGS is its wide applicability in both clinical and research settings. In clinical settings, NGS is used to diagnose various disorders, via identification of germline or somatic mutations.14,15 The shift towards NGS in clinical practice is justified by the power of the technique paired with the continually declining costs. NGS is also a valuable tool in metagenomic studies and used for infectious disease diagnostics, monitoring and management.16,17 In 2020, NGS methods were pivotal in characterizing the SARS-CoV-2 genome and are constantly contributing in monitoring the COVID-19 pandemic.18,19
Figure 1: The evolution of sequencing methodologies.
Next-generation sequencing methods
The term NGS is often taken to mean 2G technologies, however, third (3G) and fourth (4G) generation technologies have since evolved that work on different underlying principles.
Sequencing platforms/ sequencing technology
Second-generation sequencing methods are well-established and share many features in common. They can, however, be subdivided according to their underlying detection chemistries including sequencing by ligation (incorporating nanoball) and sequencing by synthesis (SBS), which further divides into proton detection, pyrosequencing and reversible terminator (Figure 2).
Figure 2: Diagram representing the principle 2G sequencing platforms and chemistries.
Proton detection sequencing relies on counting hydrogen ions released during the polymerization of DNA. Unlike other techniques, it does not use fluorescence and does not use modified nucleotides or optics. Instead, pH changes are detected by semiconductor sensor chips and converted to digital information.20
Pyrosequencing utilizes the detection of pyrophosphate generation and light release to determine whether a specific base has been incorporated in a DNA chain.21,22
By far the most popular SBS method is reversible terminator sequencing which utilizes ‘’bridge-amplification’’. During the synthesis reactions, the fragments bind to oligonucleotides on the flow cell, creating a bridge from one side of the sequence (P5 oligo on flow cell) to the other (P7), which is then amplified. The added fluorescently-labeled nucleotides are detected using direct imaging.23
Unlike SBS, sequencing by ligation does not use DNA polymerase to create a second strand. The sensitivity of DNA ligase to base-pairing mismatches is utilized instead, with the fluorescence produced used to determine the target sequence. Digital images taken after each reaction are then used for analysis. DNA nanoball sequencing is a form of sequencing by ligation that exploits rolling circle replication. Concatenated DNA copies are compacted into DNA nanoballs and bound to sequencing slides in a dense grid of spots ready for ligation-based sequencing reactions.24,25 Whilst the nanoball technique reduces running costs, the short sequences produced can be problematic for read mapping.
2G NGS technologies in general offer several advantages over alternative sequencing techniques, including the ability to generate sequencing reads in a fast, sensitive and cost-effective manner. However, there are also disadvantages, including poor interpretation of homopolymers and incorporation of incorrect dNTPs by polymerases, resulting in sequencing errors. The short read lengths also create the need for deeper sequencing coverage to enable accurate contig and final genome assembly.26–30 The major disadvantage of all 2G NGS techniques is the need for PCR amplification prior to sequencing. This is associated with PCR bias during library preparation (sequence GC-content, fragment length and false diversity) and analysis (base errors/favoring certain sequences over others).
The introduction of 3G sequencing circumvents the need for PCR, sequencing single molecules without prior amplification steps. The first single molecule sequencing (SMS) technology was developed by Stephen Quake and colleagues.31 Here, sequence information is obtained with the use of DNA polymerase by monitoring the incorporation of fluorescently labeled nucleotides to DNA strands with single base resolution. Depending on the method and the instrument used, some of the advantages of 3G NGS include:
- Real-time monitoring of nucleotide incorporation
- Non-biased sequencing and
- Longer read lengths
Nevertheless, high costs, high error rates, large quantities of sequencing data and low read depth can be problematic.32,33
In 4G systems the single-molecule sequencing of 3G is combined with nanopore technology. Similar to 3G, nanopore technology requires no amplification and uses the concept of single molecule sequencing but with the integration of tiny biopores of nanoscale diameter (nanopores) through which the single molecule passes and is identified. The 4G systems currently offer the fastest whole genome sequence scan but are still quite expensive, error prone compared to 2G techniques and relatively new. Consequently, there is currently less extensive data available for the technique.34
Main steps of 2G sequencing methods and next-generation sequencing library prep
Regardless of the 2G NGS method chosen, there are several main steps that must be tailored and optimized to the target (RNA or DNA) and sequencing system selected.
(1) Sample preparation (pre-processing)
Nucleic acids (DNA or RNA) are extracted from the selected samples (blood, sputum, bone marrow etc.). Extracted samples are quality control (QC) checked, using standard methods (spectrophotometric, fluorometric or gel electrophoretic). If using RNA, this must be reverse transcribed into cDNA, however some library preparation kits may include this step.
Random fragmentation of the cDNA or DNA, typically by enzymatic treatment or sonication, is performed. The optimal fragment length depends on the platform being used. It may be necessary to run a small amount of fragmented sample on an electrophoresis gel when optimizing this process. These fragments are then end-repaired and ligated to smaller generic DNA fragments called adapters. Adapters have defined lengths with known oligomer sequences to be compatible with the applied sequencing platform and identifiable where multiplex sequencing is performed. Multiplex sequencing, using individual adapter sequences per sample, enables large numbers of libraries to be pooled and sequenced simultaneously in a single run. This pool of DNA fragments with adapters attached are known as a sequencing library.
Size selection may then be performed, by gel electrophoresis or using magnetic beads, to remove any fragments that are too short or too long for optimal performance on the sequencing platform and protocol selected. Library enrichment/amplification is then achieved using PCR. In techniques involving emulsion PCR, each fragment is bound to a single emulsion bead which will go on to form the basis of sequencing clusters. Amplification is often followed by a “clean-up” step (e.g., using magnetic beads) to remove undesired fragments and improve sequencing efficiency.
The final libraries can undergo QC checks using qPCR, to confirm DNA quality and quantity. This will also allow the correct concentration of sample to be prepared for sequencing.
(3) Sequencing
Depending on the selected platform and chemistry, clonal amplification of library fragments may occur prior to sequencer loading (emulsion PCR) or on the sequencer itself (bridge PCR). Sequences are then detected and reported according to the platform selected.35
(4) Data analysis
The generated data files are analyzed depending on the workflow used. Analysis methods are highly dependent on the aim of the study.36–38
Whilst they may reduce the amount of samples that can be analyzed in a given run, paired-end and mate pair sequencing offer advantages in downstream data analysis, particularly for de novo assemblies. The techniques link sequencing reads together that are read from both ends of a fragment (paired-end) or are separated by an intervening DNA region (mate pair).
There are clearly many options when it comes to selecting a sequencing strategy. The following are some of the key considerations when deciding on the appropriate library preparation and sequencing platform:
(a) Research question being asked
(b) Sample type
(c) Short-read or long-read sequencing
(d) DNA or RNA sequencing – do you need to look at the genome or transcriptome?
(e) Is the whole genome required or only specific regions?
(f) Read depth (coverage) needed – experiment-specific
(g) Extraction method
(h) Sample concentration
(i) Single end, paired end or mate pair reads
(j) Specific read length required
(K) Could samples be multiplexed?
(l) Bioinformatic tools – experiment dependent. Depending on the sample and the biological question, the entire process of sequence analysis can be adapted.
Short-read vs long-read sequencing
The advantages and disadvantages of short- and long-read sequencing are summarized in Table 1.
Table 1: A table of advantages and disadvantages for short vs long read sequencing.
|
| |||
Short-read sequencing | · Higher sequence fidelity · Cheap · Can sequence fragmented DNA | · Not able to resolve structural variants, phasing alleles or distinguish highly homologous genomic regions · Unable to provide coverage of some repetitive regions | ||
Long-read sequencing | · Able to sequence genetic regions that are difficult to characterize with short-read seq due to repeat sequences · Able to resolve structural rearrangements or homologous regions · Able to read through an entire RNA transcript to determine the specific isoform · Assists de novo genome assembly | · Lower per read accuracy · Bioinformatic challenges, caused by coverage biases, high error rates in base allocation, scalability and limited availability of appropriate pipelines |
What's the difference between whole-exome vs whole-genome sequencing?
Whole genome sequencing (WGS) is the most widely used form of NGS and refers to the analysis of the entire nucleotide sequence of a genome. Whole exome sequencing (WES) on the other hand is a form of targeted sequencing that only addresses the protein coding exons. In humans, this accounts for only around 2% of the genome and consequently offers an opportunity for a greater depth of study in these regions. Due to the reduced sequencing burden, WES can also offer a more cost-effective option than WGS and reduce the volume and complexity of the resultant sequencing data. However, by sequencing only a fraction of the genome, vital information may be missed and the opportunity for novel discoveries is reduced. Despite the increased, although rapidly declining, costs and the associated data analysis challenges, WGS therefore offers a more powerful analysis that can reveal a more complete picture.
Next-generation sequencing data analysis
Any kind of NGS technology generates a significant amount of output data. The basics of sequence analysis follow a centralized workflow which includes a raw read QC step, pre-processing and mapping, followed by post-alignment processing, variant annotation, variant calling and visualization.
Assessment of the raw sequencing data is imperative to determine their quality and pave the way for all downstream analyses. It can provide a general view on the number and length of reads, any contaminating sequences, or any reads with low coverage. One of the most well-established applications for computing quality control statistics of sequencing reads is FastQC. However, for further pre-processing, such as read filtering and trimming, additional tools are needed. Trimming bases towards the ends of reads and removing leftover adapter sequences generally improves data quality. More recently, ultra-fast tools have been introduced, such as fastp, that can perform quality control, read filtering and base correction on sequencing data, combining most features from the traditional applications while also running two to five times faster than any of them alone.39
After the quality of the reads has been checked and pre-processing performed, the next step will depend on the existence of a reference genome. In the case of a de novo genome assembly, the generated sequences are aligned into contigs using their overlapping regions. This is often done with the assistance of processing pipelines that can include scaffolding steps to help with contig ordering, orientation and the removal of repetitive regions, thus increasing the assembly continuity.40,41 If the generated sequences are mapped (aligned) to a reference genome or transcriptome, variations compared to the reference sequence can be identified. Today, there is a plethora of mapping tools (more than 60), that have been adapted to handle the growing quantities of data generated by NGS, exploit technological advancements and tackle protocol developments.42 One difficulty, due to the increasing number of mappers, is being able to find the most suitable one. Information is usually scattered through publications, source codes (when available), manuals and other documentation. Some of the tools will also offer a mapping quality check that is necessary as some biases will only show after the mapping step. Similar to quality control prior to mapping, the correct processing of mapped reads is a crucial step, during which duplicated mapped reads (including but not limited to PCR artifacts) are removed. This is a standardized method, and most tools share common features. Once the reads have been mapped and processed, they need to be analyzed in an experiment-specific fashion, what is known as variant analysis. This step can identify single nucleotide polymorphisms (SNPs), indels (an insertion or deletion of bases), inversions, haplotypes, differential gene transcription in the case of RNA-seq and much more. Despite the multitude of tools for genome assembly, alignment and analysis, there is a constant need for new and improved versions to ensure that the sensitivity, accuracy and resolution can match the rapidly advancing NGS techniques.
The final step is visualization, for which data complexity can pose a significant challenge. Depending on the experiment and the research questions posed, there are a number of tools that can be used. If a reference genomes is available , the Integrated Genome Viewer (IGV)is a popular choice43, as is the Genome Browser. If experiments include WGS or WES, the Variant Explorer is a particularly good tool as it can be used to sieve through thousands of variants and allow users to focus on their most important findings. Visualization tools like VISTA allow for comparison between different genomic sequences. Programs suitable for de novo genome assemblies44 are more limited. However, tools like Bandage and Icarus have been used to explore and analyze the assembled genomes.
Next-generation sequencing bottlenecks
NGS has enabled us to discover and study genomes in ways that were never possible before. However, the complexity of the sample processing for NGS has exposed bottlenecks in managing, analyzing and storing the datasets. One of the main challenges is the computational resources required for the assembly, annotation, and analysis of sequencing data.45 The vast amount of data generated by NGS analysis is another critical challenge. Data centers are reaching high storage capacity levels and are constantly trying to cope with increasing demands, running the risk of permanent data loss.46 More strategies are continuously being suggested with the aim to increase efficiency, reduce sequencing error, maximize reproducibility and ensure correct data management.
Next-generation sequencing applications
Since the early 2000s NGS has become an invaluable tool in both research and clinical/diagnostic settings for modern medicine and in drug discovery, with the use of methods including WGS, WES, targeted sequencing, transcriptome, epigenome and metagenome sequencing dramatically increasing. Figure 3 summarizes workflows and options for targeting different datasets.
Figure 3: Flow diagram indicating possible sequencing strategies for different sample types.
Through WGS, researchers are able to study not only genes and their involvement in disease in humans and animals, but also characteristics of microbial and agricultural populations, providing important epidemiological and evolutionary data.47–52 Thus far, there has been a plethora of studies where mutations, rearrangements and fusion events were identified using WGS. Currently, WGS is used for the surveillance of antimicrobial resistance, one of the major global health challenges.53,54 As the costs are constantly decreasing, WGS is more frequently used for resequencing the entire human genome in clinical samples and may soon become routine in clinical practice.55 Ultimately, WGS will be needed to assign functionality to the remaining majority of the genome and decipher its role in diseases.
Their more focused nature make WES and targeted sequencing attractive options for population and clinical studies.56,57 Despite having more limitations as the name suggests, WES is an important clinical tool in the personalized medicine field. Genetic diagnoses for certain diseases, like cancer, as well as genetic characterization for other disorders can be achieved with this method in a more cost-effective way than WGS.
In addition to the many applications that NGS has in sequencing DNA, it can also be used for RNA analysis. This enables, for example, the genomes of RNA viruses, such as SARS and influenza, to be determined. Importantly, RNA-seq is frequently used in quantitative studies, facilitating not only the identification of transcribed genes in a DNA genome, but also the level at which they are transcribed (transcription level) according to the relative abundance of RNA transcripts. Potential rearrangements of the DNA sequences may also be identified through the identification of novel transcripts.58,59
Epigenomic sequencing allows the study of changes caused by histone modifications and DNA methylation. There are different methods employed for the study of epigenetic mechanisms, including whole genome bisulfate sequencing (WGBS), chromatin immunoprecipitation (ChIP-seq) and methylation dependent immunoprecipitation (MeDIP-seq) followed by sequencing.60,61 Depending on the selected method, the complete DNA methylome and histone modification profiles can be mapped and studied, gaining insights into genomic regulatory mechanisms.
Metagenomic sequencing can provide information for samples collected in a specific environment. It enables the comparison of differences and interactions between mixed microbial populations, as well as host responses. Some of the potential applications of metagenomic sequencing include, but are not limited to, infectious disease diagnostics and infection surveillance, antimicrobial resistance monitoring, microbiome studies and pathogen discovery.62
Technological advances in sample preparation, sequencing technologies and data analysis mean that NGS is also being used at the single cell level to study heterogeneities and rare changes in DNA, RNA and the epigenome.
Next-generation sequencing key terms and abbreviations
Table 2: Key terms and abbreviations relating to NGS.
DNA | Deoxyribonucleic acid |
RNA | Ribonucleic acid |
tRNA | Transfer ribonucleic acid |
NGS | Next-generation sequencing |
PCR | Polymerase chain reaction |
cDNA | Complementary DNA |
gDNA | Genomic DNA |
RNA-seq | RNA-sequencing |
SMS | Single molecule sequencing |
SBS | Sequencing by synthesis |
WGS | Whole genome sequencing |
WES | Whole exome sequencing |
WGBS | Whole genome bisulfate sequencing |
ChIP-seq | Chromatin immunoprecipitation sequencing |
MeDIP-seq | Methylation dependent immunoprecipitation followed by sequencing |
P5 | Primer 5 (sequencing adapter) |
P7 | Primer 7 (sequencing adapter) |
3G | Third-generation sequencing |
4G | Fourth-generation sequencing |
dNTPs | Deoxynucleoside triphosphate |
FastQC | Fast quality control |
Flow cell | Glass slide containing fluidic channels |
Library | Pool of DNA fragments with adapters attached |
Indel | Insertion or deletion of bases |
Adapters | Platform-specific sequences for fragment recognition |
fastp | Fast preprocessor |
De novo sequencing | Novel genome sequencing in the absence of a reference sequence |
Contigs | From “contiguous” - overlapping DNA fragments |
SNP | Single nucleotide polymorphism |
Scaffold | Created by linking contigs together using additional information |
SBL | Sequencing by ligation |
Paired-end | Reading a sequencing fragment from both ends and linking the data |
Mate pair | Linking sequencing reads separated by an intervening DNA region |
References
1. Watson JD, Crick FHC. Molecular structure of nucleic acids: A structure for deoxyribose nucleic acid. Nature. 1953;171(4356):737-738. doi:10.1038/171737a0
2. Franklin RE, Gosling RG. Molecular configuration in sodium thymonucleate. Nature. 1953;171(4356):740-741. doi:10.1038/171740a0
3. Holley RW, Apgar J, Everett GA, et al. Structure of a ribonucleic acid. Science (80- ). 1965;147(3664):1462-1465. doi:10.1126/science.147.3664.1462
4. Fiers W, Contreras R, Duerinck F, et al. Complete nucleotide sequence of bacteriophage MS2 RNA: Primary and secondary structure of the replicase gene. Nature. 1976;260(5551):500-507. doi:10.1038/260500a0
5. Sanger F, Nicklen S, Coulson AR. DNA sequencing with chain-terminating inhibitors. Proc Natl Acad Sci U S A. 1977;74(12):5463-5467. doi:10.1073/pnas.74.12.5463
6. Smith LM, Sanders JZ, Kaiser RJ, et al. Fluorescence detection in automated DNA sequence analysis. Nature. 1986;321(6071):674-679. doi:10.1038/321674a0
7. Hood LE, Hunkapiller MW, Smith LM. Automated DNA sequencing and analysis of the human genome. Genomics. 1987;1(3):201-212. doi:10.1016/0888-7543(87)90046-2
8. Chidgeavadze ZG, Beabealashvilli RS, Atrazhev AM, Kukhanova MK, Azhayev A V., Krayevsky AA. 2′, 3′-dideoxy-3′ amlnonudeo 5′ .triphosphates are the terminators of DNA synthesis catalyzed by DNA polymerases. Nucleic Acids Res. 1984;12(3):1671-1686. doi:10.1093/nar/12.3.1671
9. Abdellah Z, Ahmadi A, Ahmed S, et al. Finishing the euchromatic sequence of the human genome. Nature. 2004;431(7011):931-945. doi:10.1038/nature03001
10. Shendure J, Porreca GJ, Reppas NB, et al. Molecular biology: Accurate multiplex polony sequencing of an evolved bacterial genome. Science (80- ). 2005;309(5741):1728-1732. doi:10.1126/science.1117389
11. Mardis ER. Next-generation DNA sequencing methods. Annu Rev Genomics Hum Genet. 2008;9:387-402. doi:10.1146/annurev.genom.9.081307.164359
12. Shendure J, Ji H. Next-generation DNA sequencing. Nat Biotechnol. 2008;26(10):1135-1145. doi:10.1038/nbt1486
13. Mantere T, Kersten S, Hoischen A. Long-read sequencing emerging in medical genetics. Front Genet. 2019;10(MAY):426. doi:10.3389/fgene.2019.00426
14. Rizzo JM, Buck MJ. Key principles and clinical applications of “next-generation” DNA sequencing. Cancer Prev Res. 2012;5(7):887-900. doi:10.1158/1940-6207.CAPR-11-0432
15. Hartman P, Beckman K, Silverstein K, et al. Next generation sequencing for clinical diagnostics: Five year experience of an academic laboratory. Mol Genet Metab Reports. 2019;19:100464. doi:10.1016/j.ymgmr.2019.100464
16. Lefterova MI, Suarez CJ, Banaei N, Pinsky BA. Next-Generation Sequencing for Infectious Disease Diagnosis and Management: A Report of the Association for Molecular Pathology. J Mol Diagnostics. 2015;17(6):623-634. doi:10.1016/j.jmoldx.2015.07.004
17. Maljkovic Berry I, Melendrez MC, Bishop-Lilly KA, et al. Next Generation Sequencing and Bioinformatics Methodologies for Infectious Disease Research and Public Health: Approaches, Applications, and Considerations for Development of Laboratory Capacity. J Infect Dis. 2020;221(Supplement_3):S292-S307. doi:10.1093/infdis/jiz286
18. Mostafa HH, Fissel JA, Fanelli B, et al. Metagenomic next-generation sequencing of nasopharyngeal specimens collected from confirmed and suspect covid-19 patients. MBio. 2020;11(6):1-13. doi:10.1128/mBio.01969-20
19. Charre C, Ginevra C, Sabatier M, et al. Evaluation of NGS-based approaches for SARS-CoV-2 whole genome characterisation. Virus Evol. 2020;6(2):75. doi:10.1093/ve/veaa075
20. Rothberg JM, Hinz W, Rearick TM, et al. An integrated semiconductor device enabling non-optical genome sequencing. Nature. 2011;475(7356):348-352. doi:10.1038/nature10242
21. Ronaghi M, Karamohamed S, Pettersson B, Uhlén M, Nyrén P. Real-time DNA sequencing using detection of pyrophosphate release. Anal Biochem. 1996;242(1):84-89. doi:10.1006/abio.1996.0432
22. Slatko BE, Gardner AF, Ausubel FM. Overview of Next-Generation Sequencing Technologies. Curr Protoc Mol Biol. 2018;122(1):e59. doi:10.1002/cpmb.59
23. Buermans HPJ, den Dunnen JT. Next generation sequencing technology: Advances and applications. Biochim Biophys Acta - Mol Basis Dis. 2014;1842(10):1932-1941. doi:10.1016/j.bbadis.2014.06.015
24. Porreca GJ. Genome sequencing on nanoballs. Nat Biotechnol. 2010;28(1):43-44. doi:10.1038/nbt0110-43
25. Drmanac R, Sparks AB, Callow MJ, et al. Human genome sequencing using unchained base reads on self-assembling DNA nanoarrays. Science (80- ). 2010;327(5961):78-81. doi:10.1126/science.1181498
26. Liu L, Li Y, Li S, et al. Comparison of next-generation sequencing systems. J Biomed Biotechnol. 2012;2012. doi:10.1155/2012/251364
27. Peters EJ, McLeod HL. Editorial: Ability of whole-genome SNP arrays to capture ’must have pharmacogenomic variants. Pharmacogenomics. 2008;9(11):1573-1577. doi:10.2217/14622416.9.11.1573
28. Sun X, Liu D, Zhang X, et al. SLAF-seq: An Efficient Method of Large-Scale De Novo SNP Discovery and Genotyping Using High-Throughput Sequencing. Aerts J, ed. PLoS One. 2013;8(3):e58700. doi:10.1371/journal.pone.0058700
29. Timp W, Mirsaidov UM, Wang D, Comer J, Aksimentiev A, Timp G. Nanopore sequencing: Electrical measurements of the code of life. IEEE Trans Nanotechnol. 2010;9(3):281-294. doi:10.1109/TNANO.2010.2044418
30. Wang J, Lin M, Crenshaw A, et al. High-throughput single nucleotide polymorphism genotyping using nanofluidic Dynamic Arrays. BMC Genomics. 2009;10. doi:10.1186/1471-2164-10-561
31. Braslavsky I, Hebert B, Kartalov E, Quake SR. Sequence information can be obtained from single DNA molecules. Proc Natl Acad Sci U S A. 2003;100(7):3960-3964. doi:10.1073/pnas.0230489100
32. Harris TD, Buzby PR, Babcock H, et al. Single-molecule DNA sequencing of a viral genome. Science (80- ). 2008;320(5872):106-109. doi:10.1126/science.1150427
33. Roberts RJ, Carneiro MO, Schatz MC. The advantages of SMRT sequencing. Genome Biol. 2013;14(6):405. doi:10.1186/gb-2013-14-6-405
34. Thomas CS, Glassman MJ, Olsen BD. Solid-state nanostructured materials from self-assembly of a globular protein-polymer diblock copolymer. In: ACS Nano. Vol 5. ACS Nano; 2011:5697-5707. doi:10.1021/nn2013673
35. Head SR, Kiyomi Komori H, LaMere SA, et al. Library construction for next-generation sequencing: Overviews and challenges. Biotechniques. 2014;56(2):61-77. doi:10.2144/000114133
36. Goodwin S, McPherson JD, McCombie WR. Coming of age: Ten years of next-generation sequencing technologies. Nat Rev Genet. 2016;17(6):333-351. doi:10.1038/nrg.2016.49
37. Hess JF, Kohl TA, Kotrová M, et al. Library preparation for next generation sequencing: A review of automation strategies. Biotechnol Adv. 2020;41. doi:10.1016/j.biotechadv.2020.107537
38. Metzker ML. Sequencing technologies the next generation. Nat Rev Genet. 2010;11(1):31-46. doi:10.1038/nrg2626
39. Chen S, Zhou Y, Chen Y, Gu J. Fastp: An ultra-fast all-in-one FASTQ preprocessor. In: Bioinformatics. Vol 34. Oxford University Press; 2018:i884-i890. doi:10.1093/bioinformatics/bty560
40. Minei R, Hoshina R, Ogura A. De novo assembly of middle-sized genome using MinION and Illumina sequencers 06 Biological Sciences 0604 Genetics. BMC Genomics. 2018;19(1):700. doi:10.1186/s12864-018-5067-1
41. Luo J, Lyu M, Chen R, Zhang X, Luo H, Yan C. SLR: a scaffolding algorithm based on long reads and contig classification. BMC Bioinformatics. 2019;20(1):539. doi:10.1186/s12859-019-3114-9
42. Fonseca NA, Rung J, Brazma A, Marioni JC. Tools for mapping high-throughput sequencing data. Bioinformatics. 2012;28(24):3169-3177. doi:10.1093/bioinformatics/bts605
43. Robinson JT, Thorvaldsdóttir H, Winckler W, et al. Integrative genomics viewer. Nat Biotechnol. 2011;29(1):24-26. doi:10.1038/nbt.1754
44. Mikheenko A, Valin G, Prjibelski A, Saveliev V, Gurevich A. Icarus: Visualizer for de novo assembly evaluation. Bioinformatics. 2016;32(21):3321-3323. doi:10.1093/bioinformatics/btw379
45. Scholz MB, Lo CC, Chain PSG. Next generation sequencing and bioinformatic bottlenecks: The current state of metagenomic data analysis. Curr Opin Biotechnol. 2012;23(1):9-15. doi:10.1016/j.copbio.2011.11.013
46. Papageorgiou L, Eleni P, Raftopoulou S, Mantaiou M, Megalooikonomou V, Vlachakis D. Genomic big data hitting the storage bottleneck. EMBnet.journal. 2018;24:e910. doi:10.14806/ej.24.0.910
47. Wu J, Wu M, Chen T, Jiang R. Whole genome sequencing and its applications in medical genetics. Quant Biol. 2016;4(2):115-128. doi:10.1007/s40484-016-0067-0
48. Bharadwaj S, Dwivedi VD, Kirtipal N. Application of Whole Genome Sequencing (WGS) Approach Against Identification of Foodborne Bacteria. In: Microbial Genomics in Sustainable Agroecosystems. Springer Singapore; 2019:131-148. doi:10.1007/978-981-13-8739-5_7
49. Plassais J, Kim J, Davis BW, et al. Whole genome sequencing of canids reveals genomic regions under selection and variants influencing morphology. Nat Commun. 2019;10(1):1-14. doi:10.1038/s41467-019-09373-w
50. Huang X, Han B. Natural Variations and Genome-Wide Association Studies in Crop Plants. Annu Rev Plant Biol. 2014;65(1):531-551. doi:10.1146/annurev-arplant-050213-035715
51. Salipante SJ, SenGupta DJ, Cummings LA, Land TA, Hoogestraat DR, Cookson BT. Application of whole-genome sequencing for bacterial strain typing in molecular epidemiology. J Clin Microbiol. 2015;53(4):1072-1079. doi:10.1128/JCM.03385-14
52. Varshney RK, Nayak SN, May GD, Jackson SA. Next-generation sequencing technologies and their implications for crop genetics and breeding. Trends Biotechnol. 2009;27(9):522-530. doi:10.1016/j.tibtech.2009.05.006
53. Collineau L, Boerlin P, Carson CA, et al. Integrating whole-genome sequencing data into quantitative risk assessment of foodborne antimicrobial resistance: A review of opportunities and challenges. Front Microbiol. 2019;10(MAY):1107. doi:10.3389/fmicb.2019.01107
54. Hendriksen RS, Bortolaia V, Tate H, Tyson GH, Aarestrup FM, McDermott PF. Using Genomics to Track Global Antimicrobial Resistance. Front Public Heal. 2019;7:242. doi:10.3389/fpubh.2019.00242
55. Kwong JC, Mccallum N, Sintchenko V, Howden BP. Whole genome sequencing in clinical and public health microbiology. Pathology. 2015;47(3):199-210. doi:10.1097/PAT.0000000000000235
56. Suwinski P, Ong CK, Ling MHT, Poh YM, Khan AM, Ong HS. Advancing personalized medicine through the application of whole exome sequencing and big data analytics. Front Genet. 2019;10(FEB):49. doi:10.3389/fgene.2019.00049
57. Maróti Z, Boldogkoi Z, Tombácz D, Snyder M, Kalmár T. Evaluation of whole exome sequencing as an alternative to BeadChip and whole genome sequencing in human population genetic analysis 06 Biological Sciences 0604 Genetics. BMC Genomics. 2018;19(1):778. doi:10.1186/s12864-018-5168-x
58. Ozsolak F, Milos PM. RNA sequencing: Advances, challenges and opportunities. Nat Rev Genet. 2011;12(2):87-98. doi:10.1038/nrg2934
59. Wang Z, Gerstein M, Snyder M. RNA-Seq: A revolutionary tool for transcriptomics. Nat Rev Genet. 2009;10(1):57-63. doi:10.1038/nrg2484
60. Xing X, Zhang B, Li D, Wang T. Comprehensive whole DNA methylome analysis by integrating MeDIP-seq and MRE-seq. In: Methods in Molecular Biology. Vol 1708. Humana Press Inc.; 2018:209-246. doi:10.1007/978-1-4939-7481-8_12
61. Ku CS, Naidoo N, Wu M, Soong R. Studying the epigenome using next generation sequencing. J Med Genet. 2011;48(11):721-730. doi:10.1136/jmedgenet-2011-100242
62. Chiu CY, Miller SA. Clinical metagenomics. Nat Rev Genet. 2019;20(6):341-355. doi:10.1038/s41576-019-0113-7