Advances in Proteomics
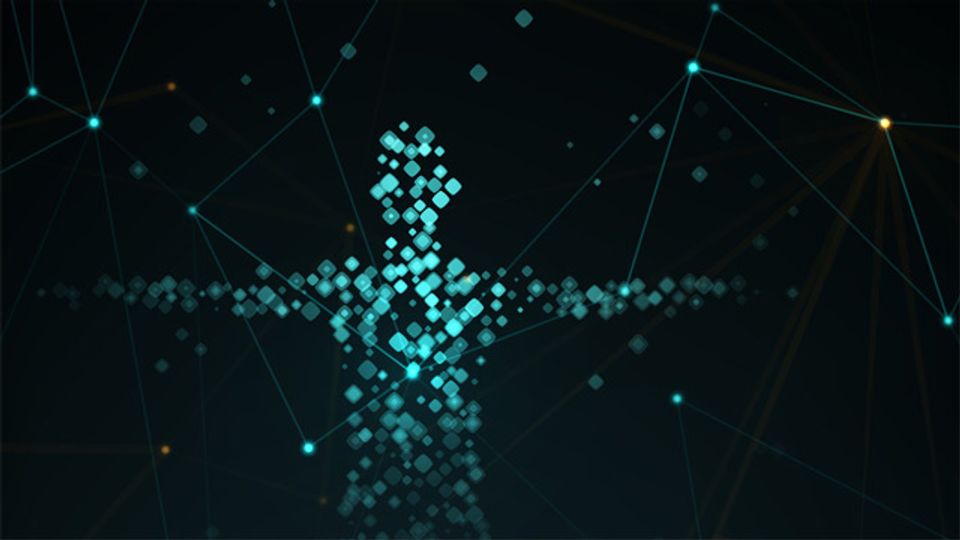
Complete the form below to unlock access to ALL audio articles.
Proteomics – the study of the proteome – is a constantly evolving field. It offers a global understanding of the molecular processes that underpin biological states across cells, tissues and whole organisms. Various areas of scientific research, including human, animal and plant biology, personalized medicine and forensics, are benefiting from rapid progress, which is largely attributed to advancements in proteomics technologies, data handling capabilities and data sharing. In this article, we will explore some of the recent advances in proteomics and their potential wider impact.
Key technological developments
Mass spectrometry proteomics
A variety of analytical techniques are adopted in proteomics research that can be broadly categorized as low- and high-throughput. For several decades, mass spectrometry (MS) has remained the most widely used “gold standard” technique for high-throughput analysis. A myriad of MS-based proteomic workflows, with unique combinations of sample preparation techniques, mass analyzers and data software pipelines, now exist. Historically, a key issue faced by MS-based proteomics has been the sensitivity and specificity of instrumentation.
The landscape of MS has changed, quite dramatically, over recent years; vendors have introduced MS instrumentation with speed, sensitivity and specificity capabilities that were previously unheard of. “We have seen a huge jump in the sensitivity of MS instrumentation and advances in the coupling of liquid chromatography with MS (LC-MS). Not long ago, proteomics studies could only characterize a few hundred of the most abundant proteins, today we can now see many thousands of proteins in relatively quick experiments,” says Dr. Harvey Johnston, a postdoctoral research scientist in Rahul Samant’s group at the Babraham Institute, Cambridge UK. Ultimately, scientists can now dig deeper into the proteome than ever before.
Professor Matthias Mann is a research director and group leader at the Proteomics Program at Novo Nordisk Foundation Center for Protein Research, director at the Max-Planck Institute of Biochemistry in Munich and one of the most highly cited scientists in the world. When asked to pinpoint a particular breakthrough in MS proteomics, Mann says, “Definitely the move towards data independent acquisition (DIA) pioneered by the Aebersold laboratory.” His thoughts are echoed by Johnston, who selects DIA – also known as sequential window acquisition of all theoretical mass spectra (SWATH-MS) – in his list of advancements that have been significant for the field.
Unlike its sister technique – data dependent analysis (DDA) – DIA fragments all precursor ions that are generated during the first cycle of tandem MS (MS1) in the second cycle (MS2), offering an unbiased analysis, larger coverage of the proteome and higher reproducibility. The use of DIA-based MS in proteomics research, particularly in the oncology space, has continued to grow over recent years. In 2019, 42 published studies focusing on several different cancer types – and utilizing various biological materials – adopted DIA-MS for proteomics analysis. DIA is also making waves in neuroscience proteomics, where it has received praise for uncovering novel information relating to Alzheimer’s disease.
Methods to accelerate DIA-based MS are continuously being explored – known as “ultra-fast” proteomics – with a recent research study confirming 43 and identifying 11 novel plasma proteome biomarkers that indicate COVID-19 severity. According to Johnston, DIA-MS is helping proteomics in its quest to reach a state of rigorous standardization: “Send an identical sample to multiple proteomics labs and you will sometimes receive quite varying results, owing to multiple workflows, instruments, analysis tools and settings, etc. However, this is improving with modern methods, especially DIA.”
Phosphorylated Peptide Post-Translational Modification Site Localization and Isomer Differentiation

Post-Translational Modification (PTM) site localization and quantification of phosphopeptides by collision induced dissociation MS/MS can be challenging, and phospho-peptides can exhibit a partial neutral loss of the phospho group. Download this app note to learn how electron activated dissociation fragmentation shows promising performances for the robust and accurate quantification of labile PTMs.
View App NoteSponsored Content
Phosphorylated Peptide Post-Translational Modification Site Localization and Isomer Differentiation

Post-Translational Modification (PTM) site localization and quantification of phosphopeptides by collision induced dissociation MS/MS can be challenging, and phospho-peptides can exhibit a partial neutral loss of the phospho group. Download this app note to learn how electron activated dissociation fragmentation shows promising performances for the robust and accurate quantification of labile PTMs.
View App NoteSponsored Content
Aptamer-based proteomics and beyond
While MS has dominated the proteomics research space for many years, a “second generation” of proteomics platforms have emerged recently that utilize aptamer-based technologies, as opposed to antibodies. Discussing such technologies, Dr. Benjamin Orsburn, researcher at Johns Hopkins University School of Medicine writes, “Although LC-MS has had a monopoly on proteomics for decades, this is clearly no longer the case.”
Aptamers are short, single stranded (ss) DNA molecules that are able to form unique confirmations, allowing them to bind selectively to biological targets, such as proteins. The technology offers specificity and selectivity that is favorable in fields such as biomarker discovery, where MS proteomics is limited by its dynamic range. “This is classically the biggest challenge to the MS biomarker discovery field, where many potential biomarkers in the blood can be far less than one trillionth the concentration of albumin,” says Johnston. “The MS will try and analyze every bit of hay to see if there is a needle, the use of antibodies or aptamers, on the other hand, can act like a magnet,” Without effective methods, albumin and other highly abundant proteins overwhelm the MS analysis.
Recent research examples utilizing aptamer-based proteomics include the identification of a protein-based signature of fibrosis (scarring of the liver) in non-alcoholic fatty liver disease (NAFLD), one of the largest causes of liver disease across the globe. Corey et al. conducted multiplex profiling across a bariatric and NAFLD cohort to identify an eight-protein panel, which distinguished various stages of NAFLD from one another.
Aptamer-based proteomics was utilized in a study of 1895 female participants from the renowned Framingham Heart Study to identify biomarkers of cardiac remodeling and incident heart failure. Seventeen proteins were found to be associated with echocardiographic traits, and six proteins were associated with incident heart failure. Further analysis utilizing genetic variant data further supported these findings.
“The use of aptamer technology appears to be less biased by the absolute protein copy number in a cell than LC-MS technology,” writes Orsburn. However, until the panels utilized are capable of identifying a higher percentage of the proteome, MS proteomics is likely to remain a preferred approach, with aptamer-based technologies utilized as a complementary method. A recently proposed aspirational protein sequencing platform that adopts barcoded DNA aptamers to recognize the terminal amino acids of peptides, attached to a next-generation sequencing chip, may offer a compromise. “The full potential of this will take time to be realized,” however, says Johnston.
The Battle Against COVID-19

The battle against COVID-19 only begins with a test to identify who is infected and who is not. To accelerate an effective response, we have to move beyond the initial diagnosis to prognosis: Download this whitepaper to learn the answers to questions such as who is at risk of getting severe disease, is there a better way to find and test candidate drugs and/or vaccines and what are the long-term effects.
View WhitepaperSponsored Content
Artificial intelligence “boosts” proteomics
Arguably one of the greatest advances in proteomics over recent years has been a “boost” provided by artificial intelligence (AI)-based methods. Machine learning, deep learning and other AI approaches are being applied at various stages of the proteomics analytical pipeline.
Artificial intelligence and drug discovery proteomics
The application of artificial intelligence (AI) to proteomics is already reshaping the drug discovery landscape. Knowing how and why specific proteins interact is imperative for advancing cell biology, developing new drugs and identifying how drugs may elicit both therapeutic and adverse effects. It’s no easy feat, however. “To understand how interacting proteins attach to each other, humans or computers have to try out all possible attachment combinations in order to find the most plausible one […] this is a very time-consuming process,” says Octavian-Eugen Ganea*, a postdoctoral researcher at the Computer Science & Artificial Intelligence Laboratory (CSAIL), Massachusetts Institute of Technology (MIT).
Further complexity arises when researchers want to capture previously unidentified interactions that could exist across a large set of proteins – like the human proteome. Ganea likens this to piecing together a large 3D puzzle. AI-based methods – particularly deep learning – offer a solution. They can accelerate the process of piecing together the 3D puzzle, which is the focus of Ganea’s research.
What is deep learning?
A subset of machine learning, deep learning comprises neural networks that simulate the behavior of the brain. These neural networks are capable of “learning” from large amounts of data.
Several commercialized protein docking approaches exist, but they rely on candidate sampling, templates and task-specific features of pre-computed meshes – all of which increase computing time. Ganea and colleagues at MIT recently published a new deep learning model – EquiDock – that takes the 3D structure of two proteins and directly identifies which areas are likely to interact. EquiDock learns to capture complex docking patterns from a large set of ~41,000 protein structures, using a geometrically constrained model with thousands of parameters that are dynamically and automatically adjusted until they solve the task. Once trained, the model was compared to four other existing docking softwares. It was capable of predicting the final protein complex in one to five seconds; a speed 80–500 times faster than the existing softwares.
“Fast computational scanning of drug side effects is one example application,” says Ganea. “This is needed in order to significantly reduce an astronomical search space that would otherwise be infeasible for all our current experimental capabilities (even world-wide aggregated).” Combining EquiDock with other protein structure prediction models, he emphasizes, would further aid drug design, protein engineering, antibody generation and mechanism of action studies, among other applications. This is an exciting prospect, Ganea says, and a “critical need” in the search for better disease treatments.
Optimizing MS-based proteomics
AI-based methods are also helping researchers to gain more insights from their data: “AI is revolutionizing what we can get out of the data,” says Mann.
MS experiments have required database searching or spectral library matching to identify proteins. This presents the opportunity for certain proteins to be incorrectly recognized or missed and is time-consuming. It has been a particular barrier for DIA MS, which relies on spectral library generation via DDA analysis. A variety of deep learning methods have now been established that are capable of predicting spectra and peptide properties. Examples include – but are not limited to – Prosit, DeepMass and more recently DeepDIA. It’s anticipated that predicted spectral libraries – capable of optimizing DIA methods – will shift the proteomics field in the direction of this approach.
Application of AI in non-MS proteomics
Outside of MS-based methods, AI is making moves in analyzing the choreography of protein movements, a research space integral to understanding pathologies characterized by tangled, clumped up proteins, like Alzheimer’s disease. Microscopy and Förster resonance energy transfer (FRET) – key methods adopted in this space – generate large data sets that require time and expertise to analyze. To overcome this data slog, researchers at the Novo Nordisk Foundation Center for Protein Research, led by Professor Nikos Hatzakis, recently created DeepFRET. DeepFRET is a machine learning algorithm that recognizes protein movement patterns, classifying data sets within seconds, compared to several days’ worth of work that is typically required.
The future of AI in proteomics will require synchronicity across groups in terms of the standards that AI platforms must uphold, data reporting and sharing. Official recommendations, such as the recently published Data, Optimization, Model, Evaluation (DOME) recommendations for both conducting and reporting on machine learning in proteomics and metabolomics, will likely help to shape the field going forward.
Broader applications
There are broader applications of proteomics which also benefit from the technological advances discussed previously, such as forensic science. The “DNA revolution” that occurred in the latter part of the twentieth century dramatically transformed the field. Now, proteomics looks poised to have a similar impact. Dr. Glendon Parker, adjunct associate professor in the Department of Environmental Toxicology at UC Davis, and the inventor of Protein-Based Human Identification says that, overall, the current impact of proteomics on forensic science is limited, attributed to technical, legal, financial and cultural factors. However, “There is a fundamental drive to adopting and incorporating new methods in criminal investigation and prosecution. Proteomics has intrinsic advantages: proteins are more stable than DNA, and like DNA, can contain identifying information,” he adds.
In cases where nucleic acids have been degraded, proteomics can be utilized to identify body fluids, gender, ethnic group and to estimate the approximate time of death using muscle, bone and decomposition fluid samples.
Parker emphasizes that, while implementation has been a challenge, “In the future proteomics has the potential to significantly change how evidence is processed and analyzed. In the short term however, the DNA-centric aspect of the field ensures that proteomics will be used in areas where DNA struggles to provide clear, easily defensible answers.”
Challenges and future perspectives
Democratizing proteomics
Arguably the greatest limitation faced by the proteomics field has been its intricacy. Proteomics workflows comprise complex technologies and softwares that require skilled personnel to operate. While incredible gains in sensitivity and speed have progressed research, they come at a cost. “Rigorously executed, deep-coverage MS experiments, especially on complex biological samples require significant MS time,” says Johnston. “So, there are constantly trade-offs between cost, coverage and sample numbers.”
It's an issue that limits the broader applications of proteomics. Discussing forensic science specifically, Parker says, “Ultimately, these factors combine to restrict innovation generally, and promising emerging technologies, including proteomics, are under-utilized.”
Over the last decade, calls for a “democratization” in proteomics have increased. A number of initiatives have emerged to increase accessibility and sustainability. One example is The European Proteomics Infrastructure Consortium providing access (EPIC-XS) consortium. The initiative unites some of Europe’s leading laboratories and scientists to pool together various technologies, expertise and data sharing.
Resources aren’t limited to just MS-based proteomics. Expertise is also offered by the Cell Profiling facility at the KTH Access Site in antibody-based imaging. “This arsenal of techniques ensures the EPIC-XS platform is well equipped to consider applications from users across the diverse field of proteomics,” says Martina O’Flaherty, project manager at Utrecht University.
The road to the clinic
Before proteomics is established as a mainstay in the clinic, there are several challenges to overcome that vary depending on the particular sub-application of clinical proteomics being discussed.
“MS-based proteomics needs to become even more robust and accessible, especially if it is to be employed in the clinic at scale,” says Mann. “A number of groups have turned to high flow chromatographic systems to achieve this, but this is not ideal because sensitivity suffers.” While analytical technologies increase in capabilities to dig deeper into the proteome, the amount of data generated also grows, introducing an additional bottleneck for clinical proteomics in the form of data handling and formulating biological and clinical hypotheses from such large data sets.
Furthermore, for a holistic understanding of human health and disease, proteomics data must often be integrated with other “omics” counterparts, like metabolomics, genomics and transcriptomics.
Ethical considerations must also be considered as proteomics edges towards the clinic. Proteomic profiling can offer information outside of the original diagnostic query that instigated the test. How do clinicians handle such data? While lessons can be learned from the implementation of clinical genomics, the fields are distinct and this must be acknowledged in the development of regulatory frameworks and guidelines, experts emphasize.
Despite current limitations, Mann believes that the future of the field looks bright: “I foresee a continued move towards clinical applications where the inherent specificity of MS detection is invaluable,” he concludes.
*Technology Networks is deeply saddened to learn of Dr. Octavian-Eugen Ganea’s recent passing. We would like to acknowledge his incredible contributions to the scientific community and his dedication to the field of computational biology. Our thoughts are with those affected by his loss.